Published Research
Interpretable ML Models For Clinical Decision-Making
Oak Street Health, a network of more than 80 primary care centers in medically underserved communities, successfully implemented a machine learning-based risk stratification tool that outperformed prior backward-looking approaches in identifying high-risk patients.
While healthcare organizations are increasingly interested in using artificial intelligence (AI), there is a significant lack of literature on the real-world application of risk stratification AI tools in primary care. Oak Street Health, a network of more than 80 primary care centers in medically underserved communities, successfully implemented a machine learning-based risk stratification tool that outperformed prior backward-looking approaches in identifying high-risk patients.
The data science team collaborated with an interdisciplinary set of stakeholders to test, iterate, and implement the tool into clinical practice. Early feedback from Oak Street Health’s primary care providers (physicians and nurse practitioners) and non-providers (social workers) suggests that the display of top risk factors based on model predictions created a broadly interpretable and actionable risk stratification tool in caring for the highest-risk patients.
Download the Published Research Paper to Learn More
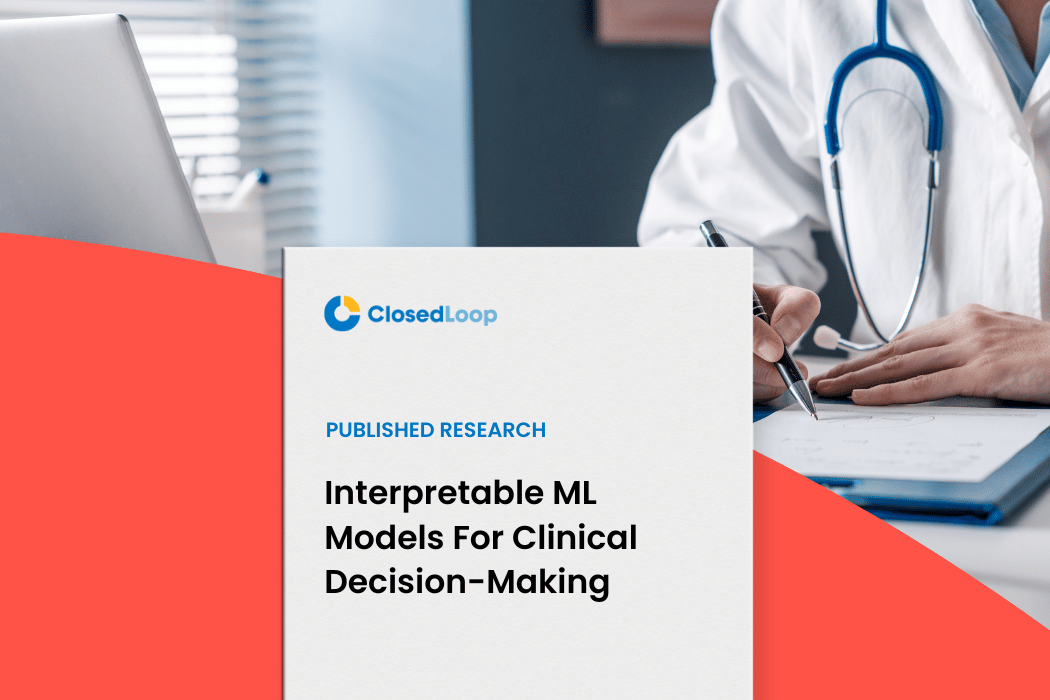
Read the paper
Related Resources
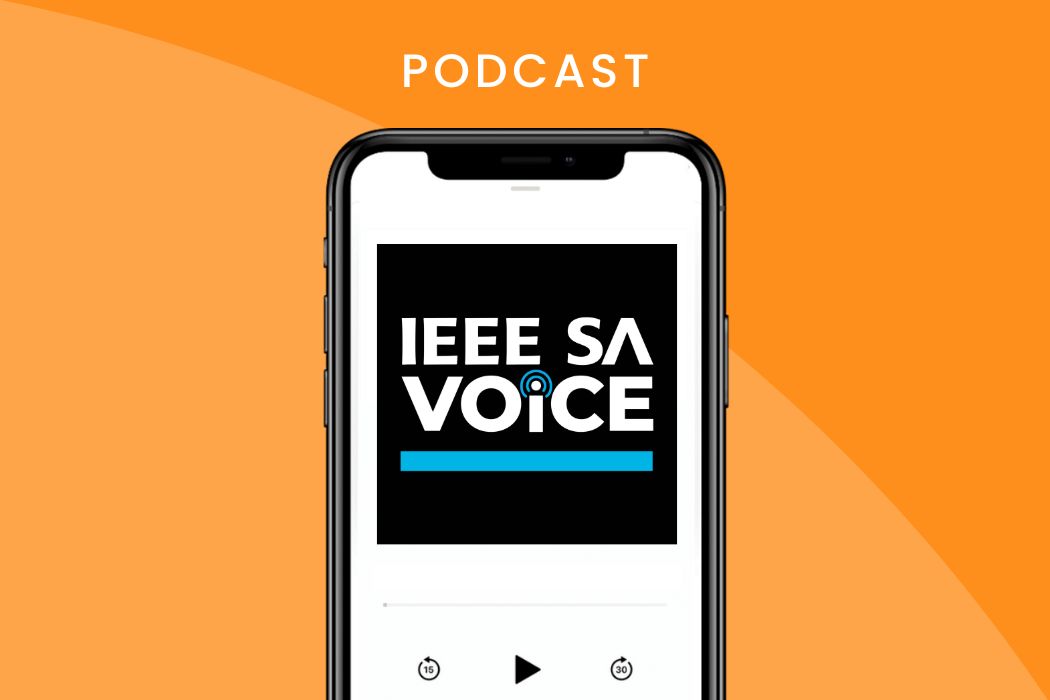
Reducing the Healthcare Gap with Explainable AI
Dave DeCaprio, CTO & Co-Founder, and Maria Palombini, Director of the IEEE SA Healthcare and Life Sciences global practice, discuss how AI presents a new perspective on transparency, reduction of bias, and a path toward health stakeholders’ trust with explainability in its applications.
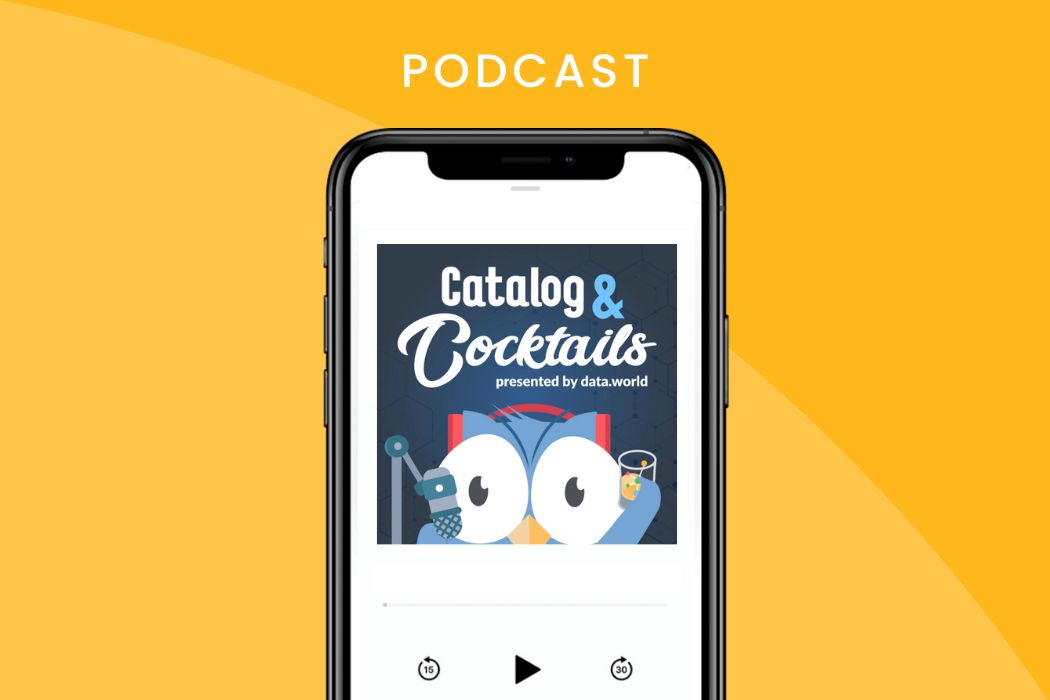
AI: No One Wants Your Models
Tim Gasper, Juan Sequeda, and Andrew Eye, CEO of ClosedLoop, discuss the challenges of AI deployment, AI Ops, and maintaining models as data changes.
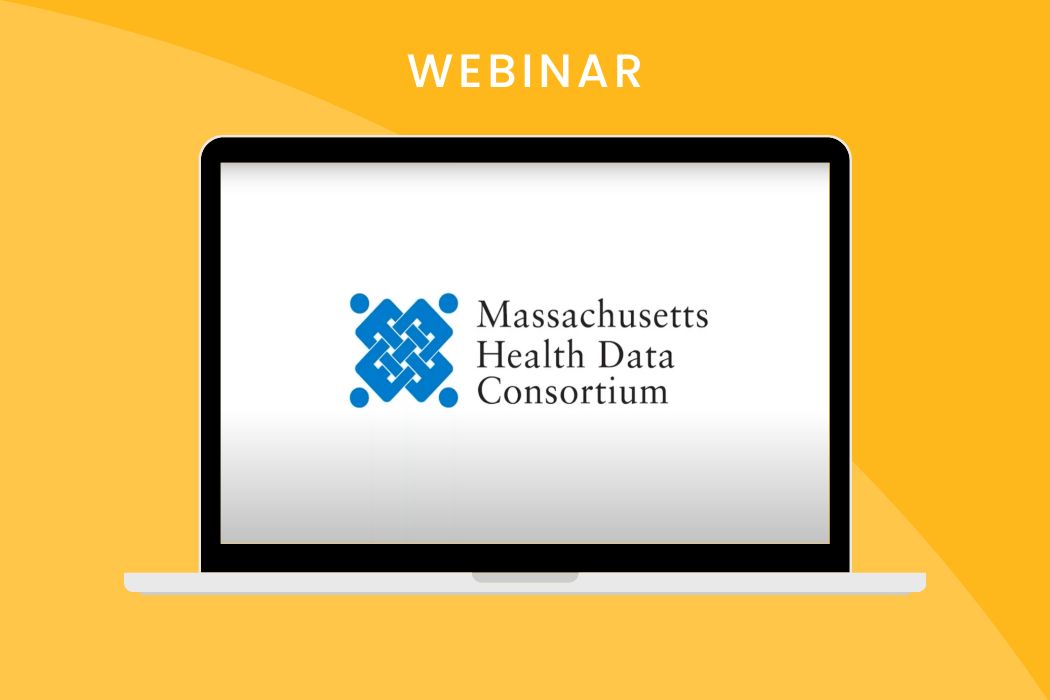
AI = ROI How AI Drives Health Outcomes and Tangible ROI in Healthcare
In this webinar with Massachusetts Health Data Consortium, ClosedLoop discusses measuring tangible ROI for predictive systems, creating explainable AI, addressing algorithmic bias, and overcoming the deployment challenges of machine learning models.