White Papers
Why Most Fairness Metrics Don’t Work in Healthcare AI/ML
Selecting an appropriate definition of fairness is difficult for healthcare algorithms, as they are applied to myriad diverse problems. Read the paper to learn why we need different definitions of fairness and to understand the most ideal fairness metric for population health AI/ML.
While most existing fairness metrics aren’t appropriate to assess algorithms that inform population health decisions, healthcare organizations still have a responsibility to ensure their algorithms help fairly distribute limited resources. Learn about a new fairness metric developed to address the unique challenges of assessing fairness in a healthcare setting: Group Benefit Equality (GBE). With GBE, healthcare organizations now have a fairness metric to ensure that their predictive models reduce health disparities rather than exacerbate them.
Read the paper to learn:
- The existing problem with measuring fairness
- What an appropriate fairness metric must address
- About Group Benefit Equality (GBE), the most ideal fairness metric for population health AI/ML
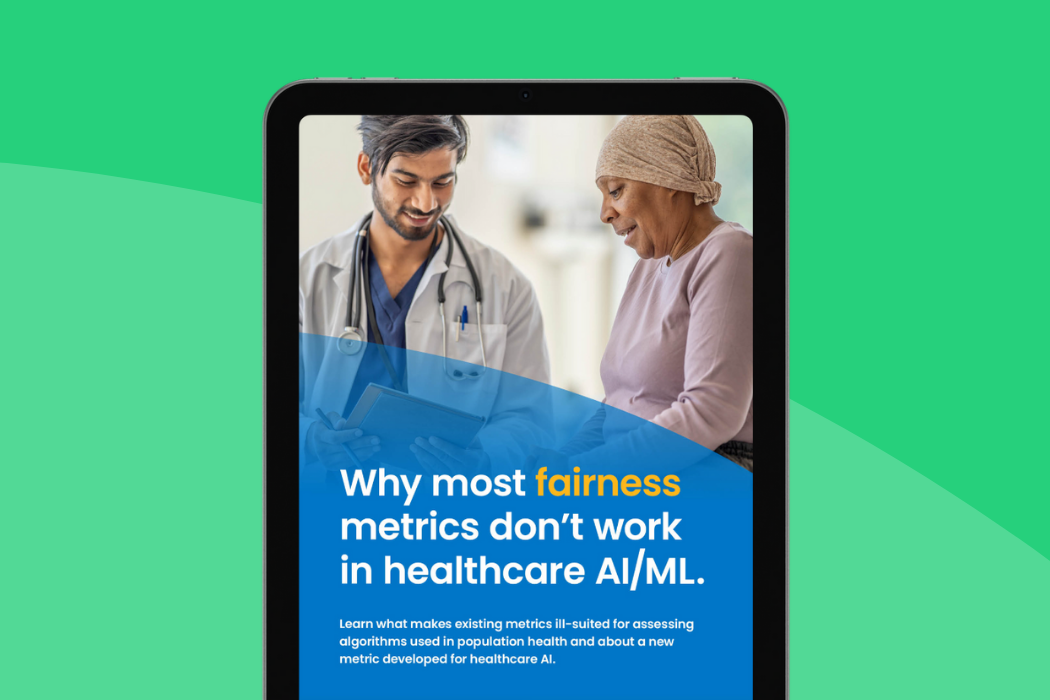
Read the white paper
Related Resources
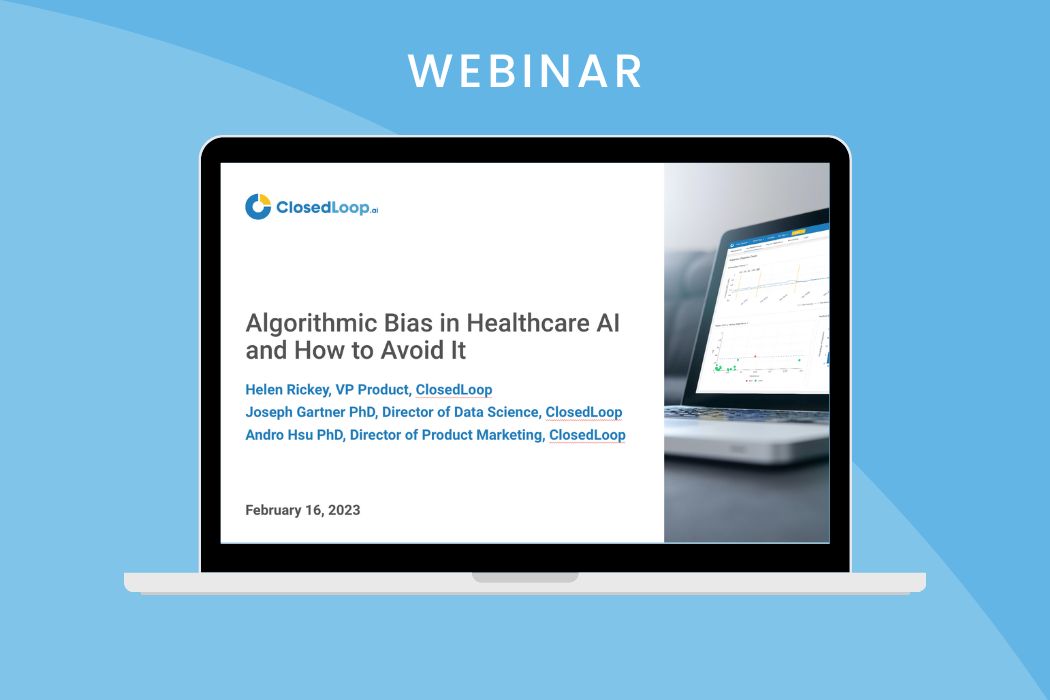
Algorithmic Bias in Healthcare AI and How to Avoid It
In this AIMed webinar, ClosedLoop explains why assessing algorithmic bias is critical for ensuring healthcare resources are fairly allocated to members. Tune in as AIMed hosts an important conversation about avoiding hidden bias in healthcare AI.
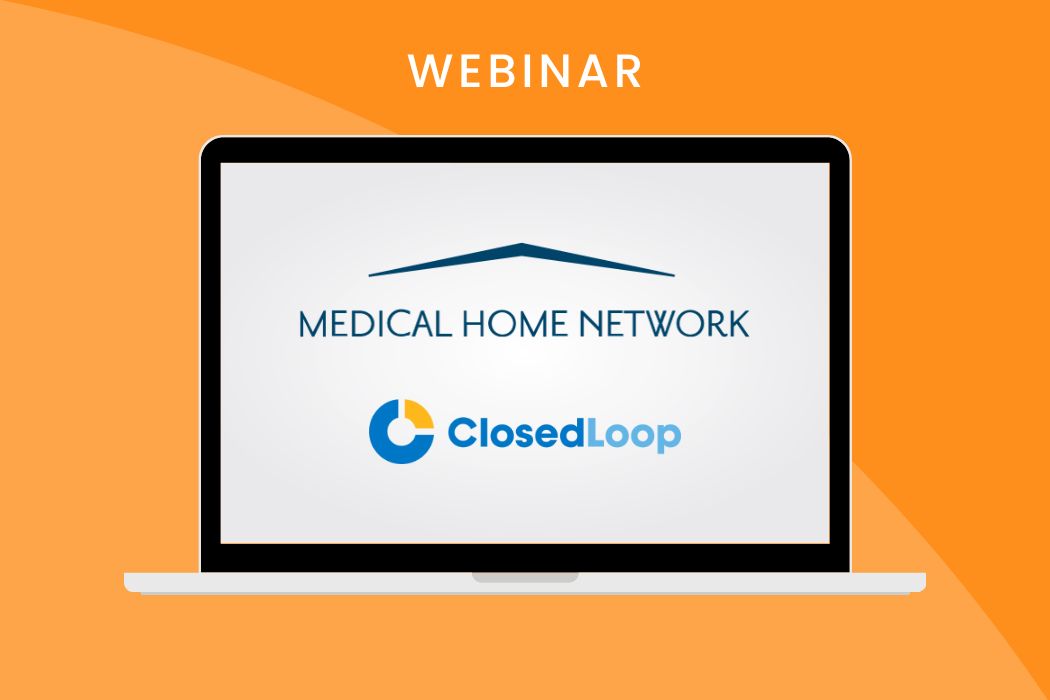
How and Why You Should Assess Bias & Fairness in Healthcare AI Before Deploying to Clinical Workflows
Watch the on-demand session to learn why it's important to evaluate algorithms for bias before deployment and what metrics you can use to assess bias. Plus, get a demo of new product features built precisely for this purpose.
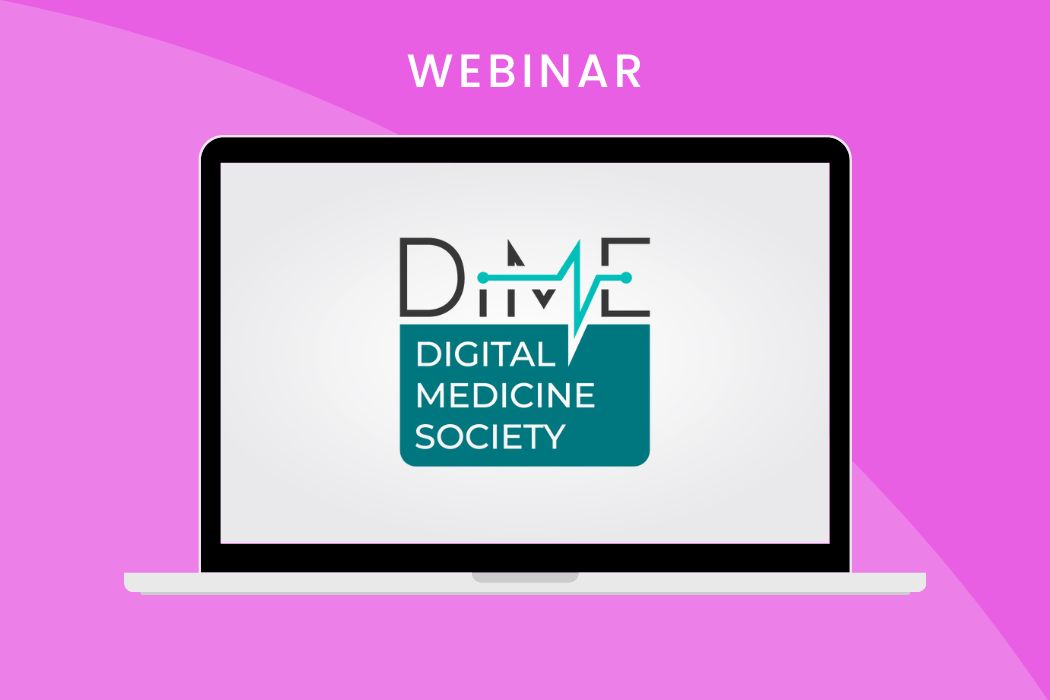
DiME Webinar: Addressing Bias in the Evolving Landscape of AI in Health Care
This on-demand webinar explores the role of bias related to the use of data for AI in healthcare solutions and how clinicians, tech companies, and other stakeholders are learning mitigation approaches as the AI landscape evolves.