Predict | Adverse Glycemic Events & Continuous Glucose Monitoring
Improve glycemic control and avoid adverse outcomes.
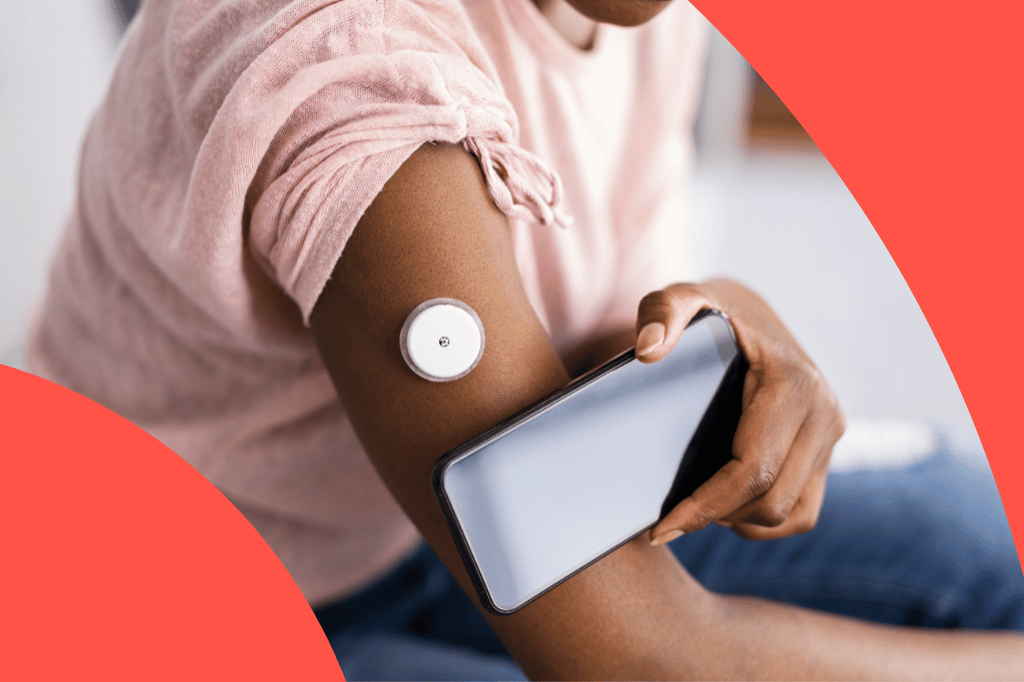
Deficient glycemic control often leads to adverse glycemic events and unplanned admissions. This contributes substantially to the burden of diabetes, which affects 34 million U.S. adults at a total estimated cost of $327 billion annually. Continuous glucose monitoring presents a key opportunity to improve glucose management for insulin intensive type 2 diabetics and avoid these adverse events.
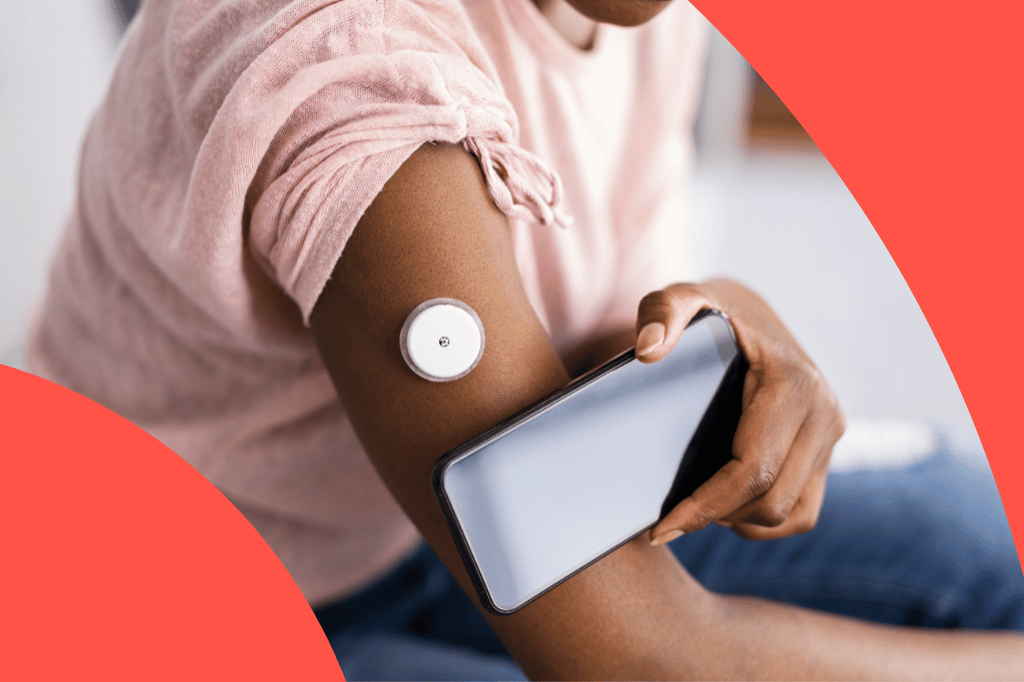
BUILT FOR HEALTHCARE
Ingest, normalize, and blend data
from dozens of health data sources.
Electronic Health Records
Unstructured Clinical Notes
e-Prescribing Data
Vital Signs
Remote Monitoring Data
Medical Claims
Rx Claims
ADT Records
Lab Test Results
Social Needs Assessments
Social Determinants of Health
Operations & Services
Risk of hypoglycemia-related ED visit in the next 6 months
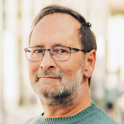
Patient ID
Gender
Age
Risk Score Percentile
874665101
Male
54
97
Impact on risk
Contributing factor
Value
# of Hypoglycemic Plasma Glucose Concentration Readings
2
Daily Insulin Use
225 units / day
HbA1C Level (3M)
8.8%
# of Unplanned Admissions (12M)
1
AI INFORMS ACTION
Pinpoint high-risk individuals and surface actionable risk factors.
ClosedLoop generates explainable predictions using thousands of auto-generated, clinically relevant contributing factors.
Employ
Employ CGM devices to improve glucose management for high-risk patients
Evaluate
Evaluate and refine insulin-based therapy with patient-specific insights
Enhance
Enhance monitoring to anticipate and avoid adverse events
EXPLORE MORE USE CASES