Predict | Appointment No-Shows
Predict appointment no-shows and reduce costs.
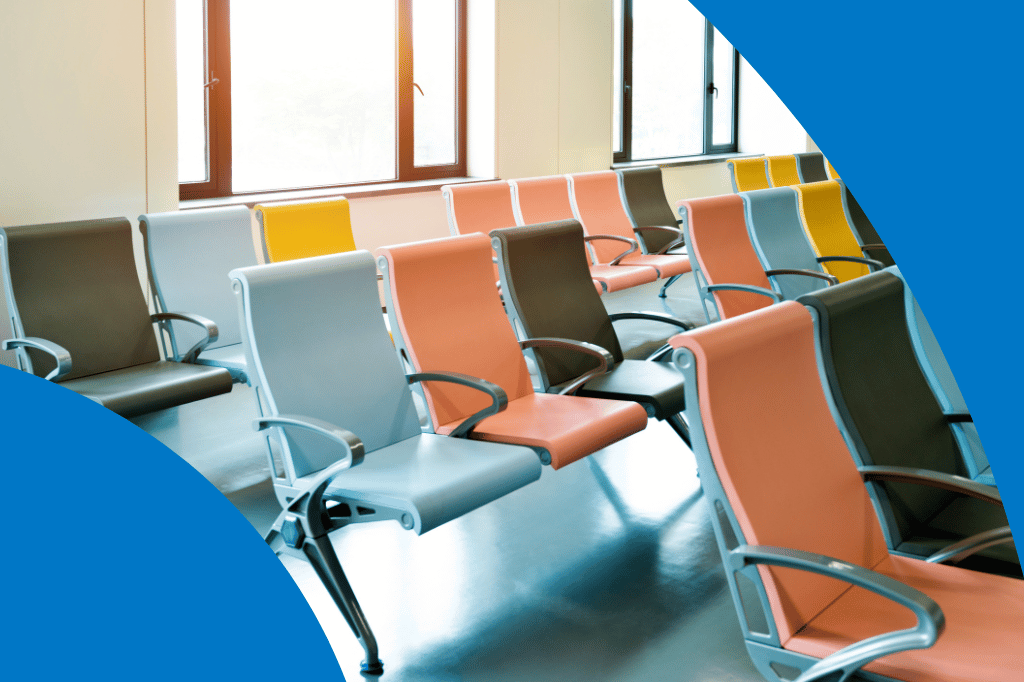
Nationally, patients are “no-shows” for 30% of their scheduled appointments, representing a $150 billion financial burden on the healthcare system annually. In addition to financial strain, missing appointments can lead to poor continuity of care, increased acute care utilization, and declines in health that could have been mitigated or prevented with earlier diagnosis and treatment.
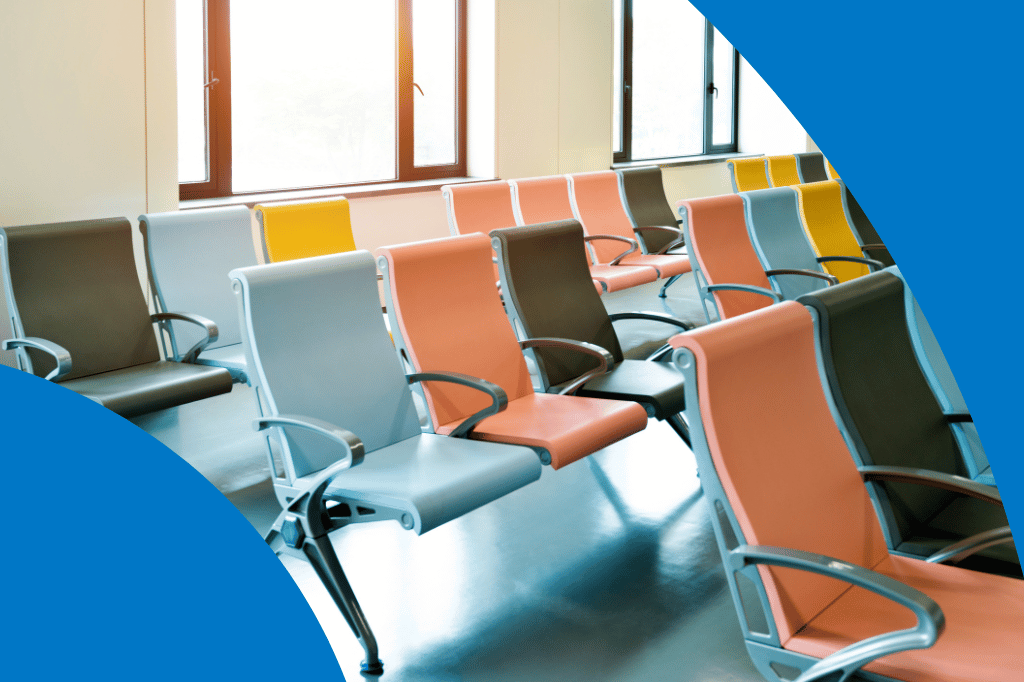
BUILT FOR HEALTHCARE
Ingest, normalize, and blend data
from dozens of health data sources.
Electronic Health Records
Unstructured Clinical Notes
e-Prescribing Data
Vital Signs
Remote Monitoring Data
Medical Claims
Rx Claims
ADT Records
Lab Test Results
Social Needs Assessments
Social Determinants of Health
Operations & Services
Risk of not showing for a scheduled appt in the next six months
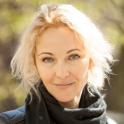
Patient ID
Gender
Age
Risk Score Percentile
311098786
Female
43
98
Impact on risk
Contributing factor
Value
# of Missed / Rescheduled Appts (12M)
4
Reported Barriers to Care
Transportation
# of Unique Prescribers (12M)
4
Low Medication Adherence
40%
AI INFORMS ACTION
Pinpoint high-risk individuals and surface actionable risk factors.
ClosedLoop generates explainable predictions using thousands of auto-generated, clinically relevant contributing factors.
Target
Target outreach and send reminders to likely no-shows
Ensure
Ensure access to reliable, timely transportation
Educate
Educate patients about the impact appointments have on achieving health goals
EXPLORE MORE USE CASES