Predict | End of Life
Predict mortality risk and improve end‑of‑life care.
Patients in the last days of life can experience unrelieved physical suffering as well as significant emotional, spiritual, and social distress. Unfortunately, they are frequently not recognized as dying. More than one in three people that prefer palliative care do not receive it, and these patients incur 1.4x the costs of patients who receive end-of-life care consistent with their wishes.
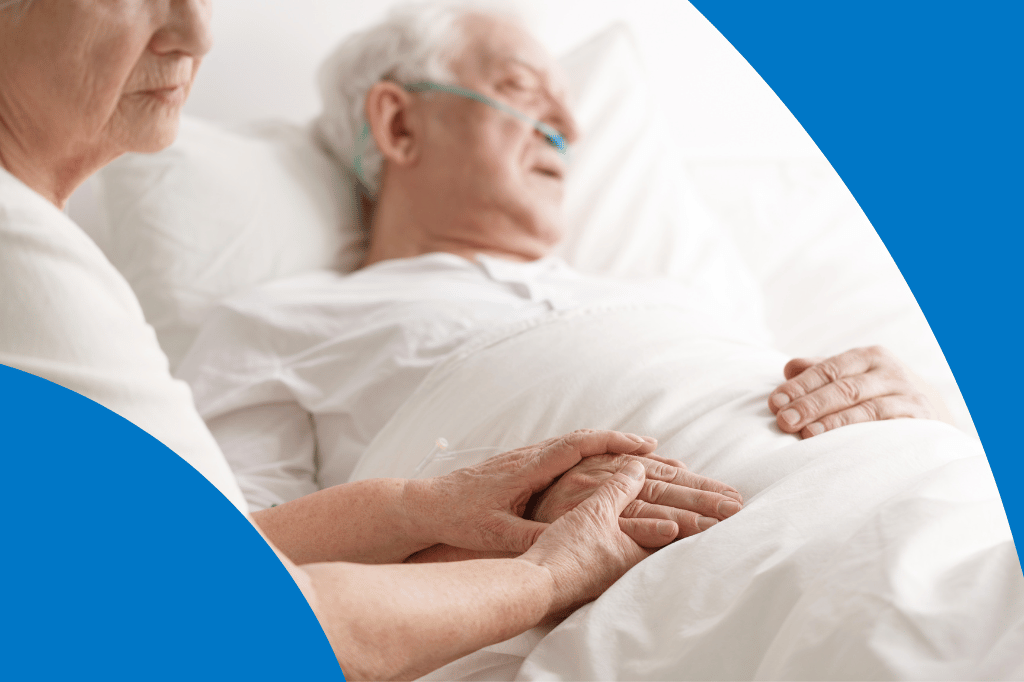
BUILT FOR HEALTHCARE
Ingest, normalize, and blend data
from dozens of health data sources.
Electronic Health Records
Unstructured Clinical Notes
e-Prescribing Data
Vital Signs
Remote Monitoring Data
Medical Claims
Rx Claims
ADT Records
Lab Test Results
Social Needs Assessments
Social Determinants of Health
Operations & Services
Risk of all-cause mortality in the next 12 months
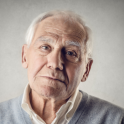
Patient ID
Gender
Age
Risk Score Percentile
223451789
Male
75
93
Impact on risk
Contributing factor
Value
Increase in Charlson Comorbidity Index
8 to 12
Diagnosis of Chronic Skin Ulcers (12M)
3
Admission for Serious Infection (3M)
1
Levels of Caregiver Support
Low
AI INFORMS ACTION
Pinpoint high-risk individuals and surface actionable risk factors.
ClosedLoop generates explainable predictions using thousands of auto-generated, clinically relevant contributing factors.
Provide
Provide palliative care to assuage symptoms and improve quality of life
Discuss
Discuss serious illness care goals
Ensure
Ensure social and emotional support for patients in their final days
EXPLORE MORE USE CASES