Predict | Heart Failure
Predict heart failure risk and reduce readmissions.
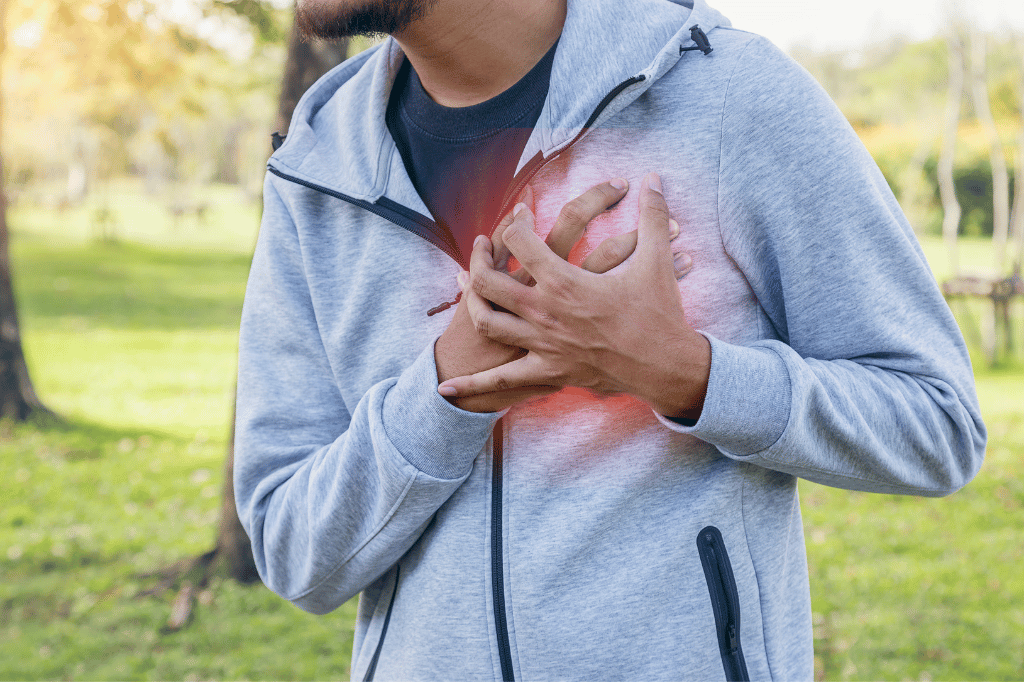
Approximately 6.5 million adult Americans are living with heart failure (HF). There are more than one million HF hospitalizations each year, and it is one of the most common causes of hospitalization, readmission, and death. Only 10% of all FFS Medicare beneficiaries have HF, yet their costs are 33% of all Medicare costs. The economic impact associated with HF will exceed $70 billion in 2030.
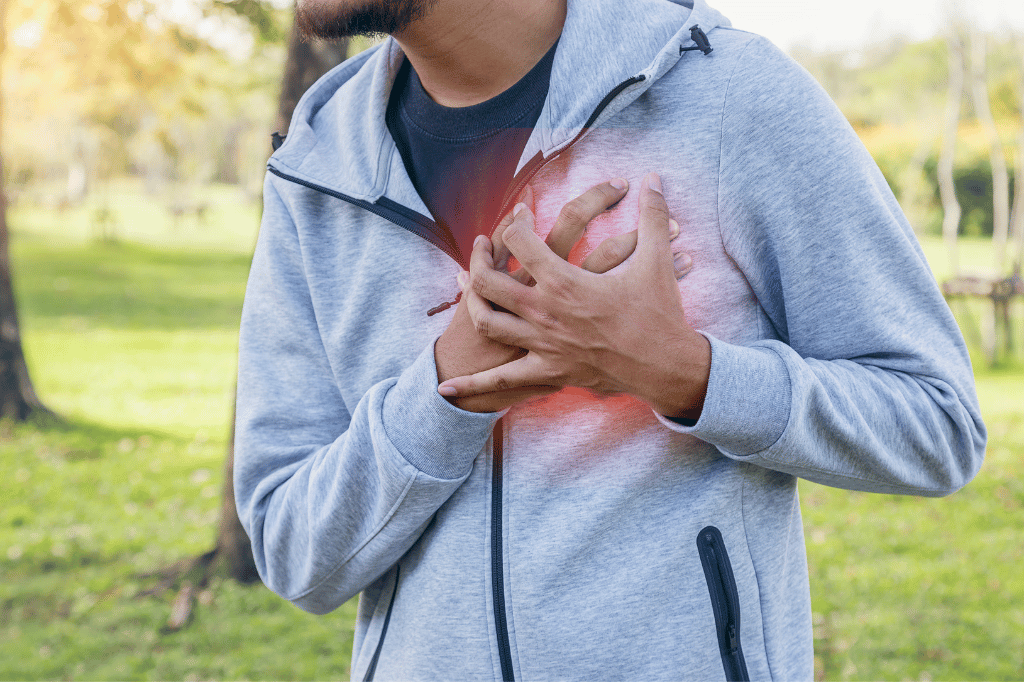
BUILT FOR HEALTHCARE
Ingest, normalize, and blend data
from dozens of health data sources.
Electronic Health Records
Unstructured Clinical Notes
e-Prescribing Data
Vital Signs
Remote Monitoring Data
Medical Claims
Rx Claims
ADT Records
Lab Test Results
Social Needs Assessments
Social Determinants of Health
Operations & Services
Risk of admission for acute heart failure in the next 12 months
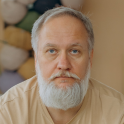
Patient ID
Gender
Age
Risk Score Percentile
134985712
Male
62
98
Impact on risk
Contributing factor
Value
# of Acute Decompensated HF Admissions (12M)
2
Decline in LV Ejection Fraction
0.45 to 0.35
Adherence to Loop Diuretics
60%
Hemoglobin (g/dL)
11.5
AI INFORMS ACTION
Pinpoint high-risk individuals and surface actionable risk factors.
ClosedLoop generates explainable predictions using thousands of auto-generated, clinically relevant contributing factors.
Encourage
Encourage strong continuity of care, promote planned visits, and engage in team-based care
Maintain
Maintain adherence to dietary restrictions and complex medication regimes
Teach
Teach patient self-management skills to reduce readmissions
EXPLORE MORE USE CASES