Predict | Length of Stay
Reduce length of stay and improve outcomes.
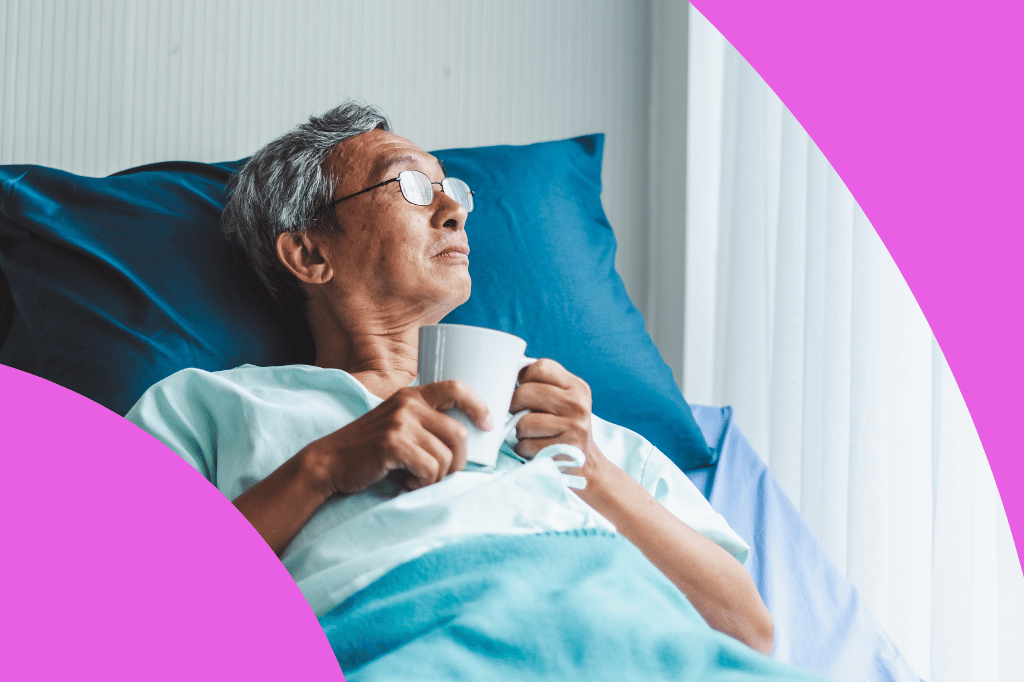
Every year, there are more than 35.7 million hospital stays in the U.S., totaling over $415 billion in annual healthcare spending. The average length of stay (LOS) is 4.6 days. If it can be safely reduced, in addition to curbing excess spending, eliminating unnecessary hospital days has the potential to significantly improve patient health outcomes.
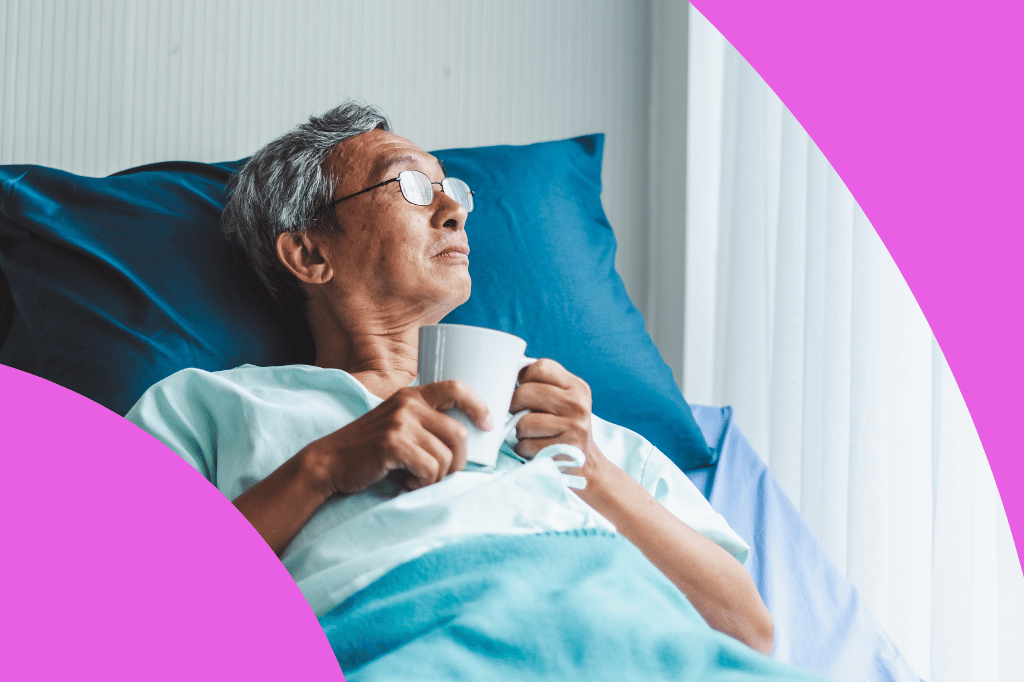
BUILT FOR HEALTHCARE
Ingest, normalize, and blend data
from dozens of health data sources.
Electronic Health Records
Unstructured Clinical Notes
e-Prescribing Data
Vital Signs
Remote Monitoring Data
Medical Claims
Rx Claims
ADT Records
Lab Test Results
Social Needs Assessments
Social Determinants of Health
Care Quality
Risk of HAI if admitted in the next 6 months
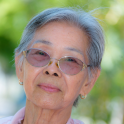
Patient ID
Gender
Age
Risk Score Percentile
114908766
Female
78
94
Impact on risk
Contributing factor
Value
Diagnosis of Bacterial Infection (12M)
Dec 2020
Diagnosis of Diabetes (12M)
1
# of ER Visits (6M)
2
30-Day Hospital Readmit Rate
0.27
AI INFORMS ACTION
Pinpoint high-risk individuals and surface actionable risk factors.
ClosedLoop generates explainable predictions using thousands of auto-generated, clinically relevant contributing factors.
Enhance
Enhance resource management planning
Optimize
Optimize hand-off procedures and communication
Accelerate
Accelerate the discharge process
EXPLORE MORE USE CASES
Admission to a Skilled Nursing Facility
Predict SNF admission risk and reduce rehospitalization.