Predict | Maternal Health and Obstetric Outcomes
Enhance maternal care and avoid adverse outcomes.
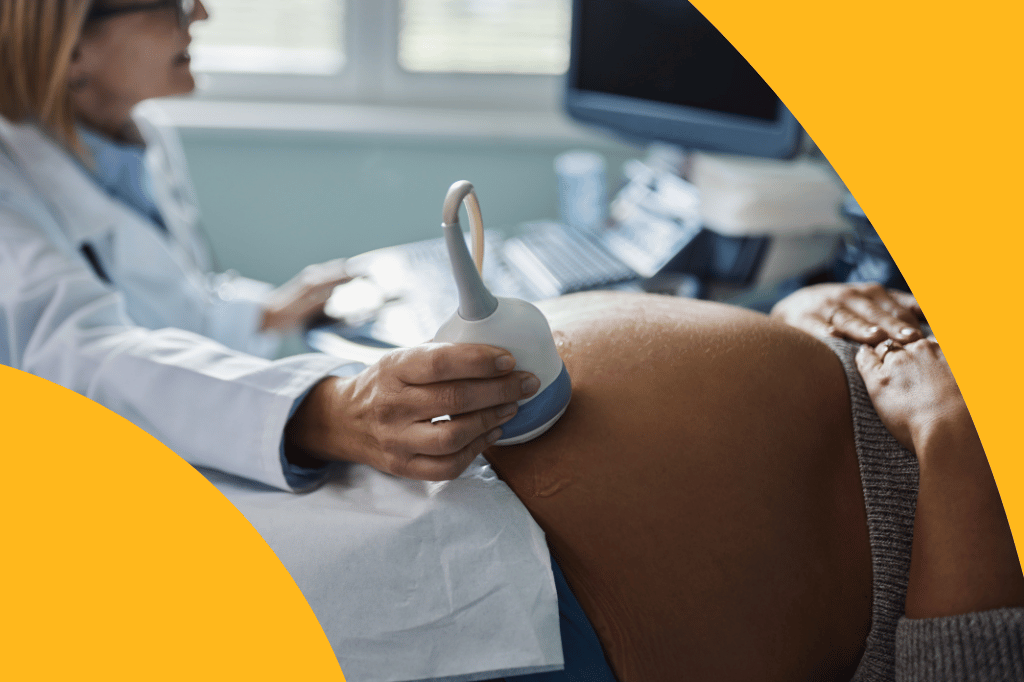
The 2020 U.S. maternal mortality rate ranked last among all industrialized countries, and more than 60,000 women suffer from severe maternal morbidity annually. Maternal health data also evinces immense racial and ethnic disparities. Black women are three to four times more likely to die a pregnancy-related death compared to White women. Reducing these disparities is key to improving obstetric outcomes.
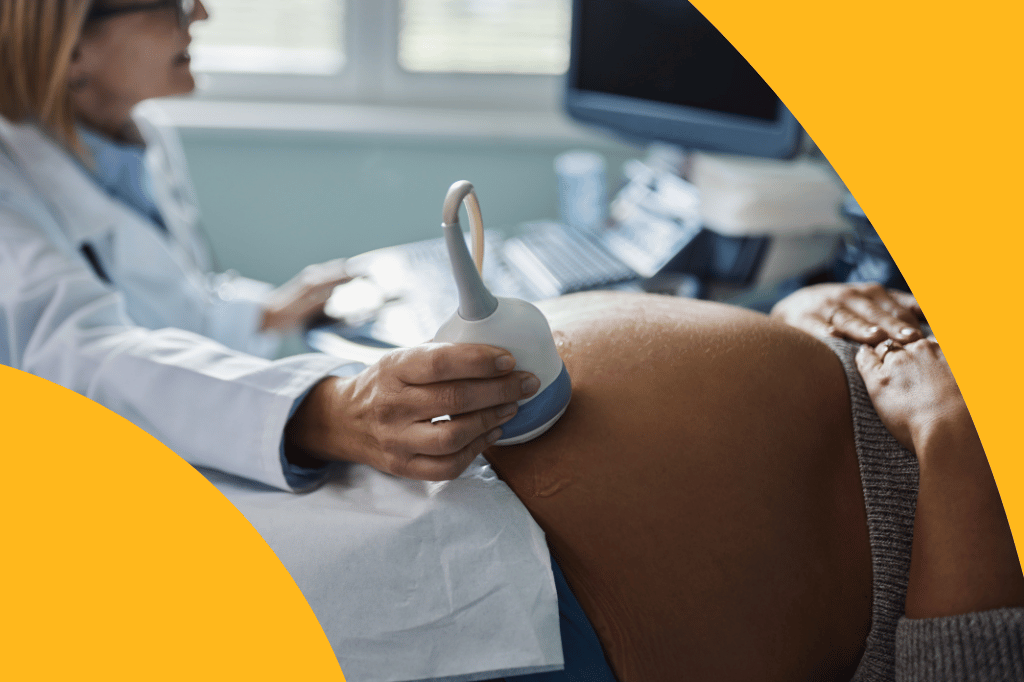
BUILT FOR HEALTHCARE
Ingest, normalize, and blend data
from dozens of health data sources.
Electronic Health Records
Unstructured Clinical Notes
e-Prescribing Data
Vital Signs
Remote Monitoring Data
Medical Claims
Rx Claims
ADT Records
Lab Test Results
Social Needs Assessments
Social Determinants of Health
Operations & Services
Risk of preeclampsia-related preterm birth in the next 3 months
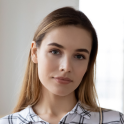
Patient ID
Gender
Age
Risk Score Percentile
287503934
Female
37
94
Impact on risk
Contributing factor
Value
# of Prenatal Care Visits (6M)
0.0
Rise in Blood Pressure (mmHg)
130/80 to 145/90
Diagnosis of Diabetes (12M)
2
Eligible for Full Medicaid Benefits
Yes
AI INFORMS ACTION
Pinpoint high-risk individuals and surface actionable risk factors.
ClosedLoop generates explainable predictions using thousands of auto-generated, clinically relevant contributing factors.
Target
Target individuals for enrollment in midwifery-led care in birth centers
Enhance
Enhance prenatal care and address gaps in the maternal care continuum
Address
Address psychosocial needs and refer to pregnancy-related resources
EXPLORE MORE USE CASES
Adverse Glycemic Events & Continuous Glucose Monitoring
Improve glycemic control and avoid adverse outcomes.