Predict | Metabolic Syndrome
Identify metabolic syndrome and promote early detection.
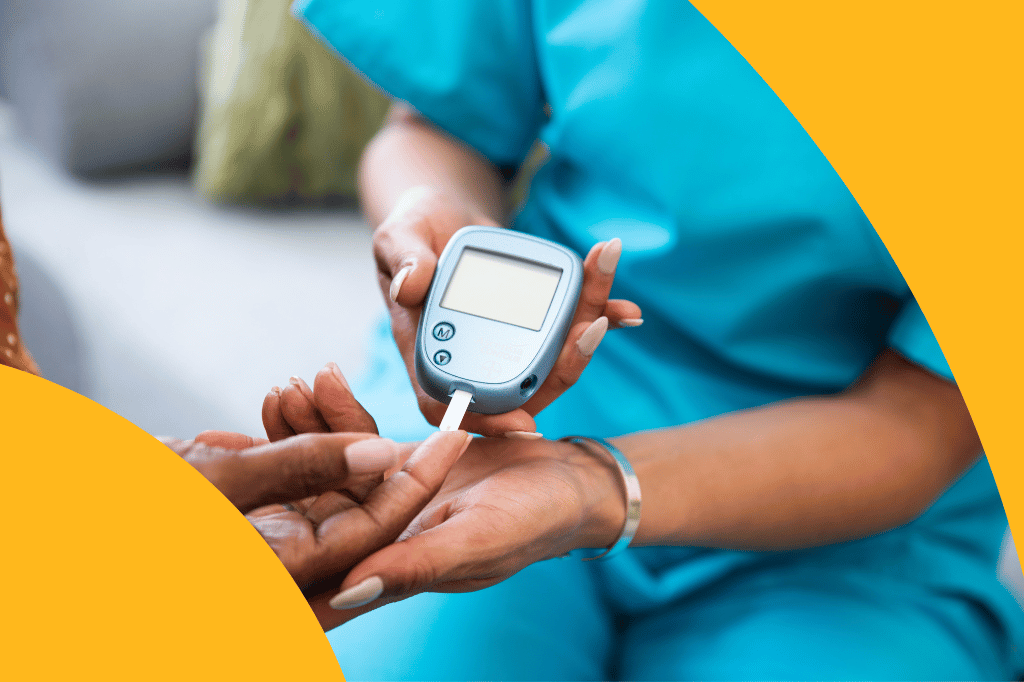
Almost one-third of all U.S. adults—approximately 80 million people—meet the criteria for metabolic syndrome (MetS), a clustering of risk factors that often leads to chronic diseases. And yet, public awareness of MetS is alarmingly low. This is especially problematic since it can be mitigated and prevented, improving outcomes and lowering the $220 billion associated annual healthcare costs.
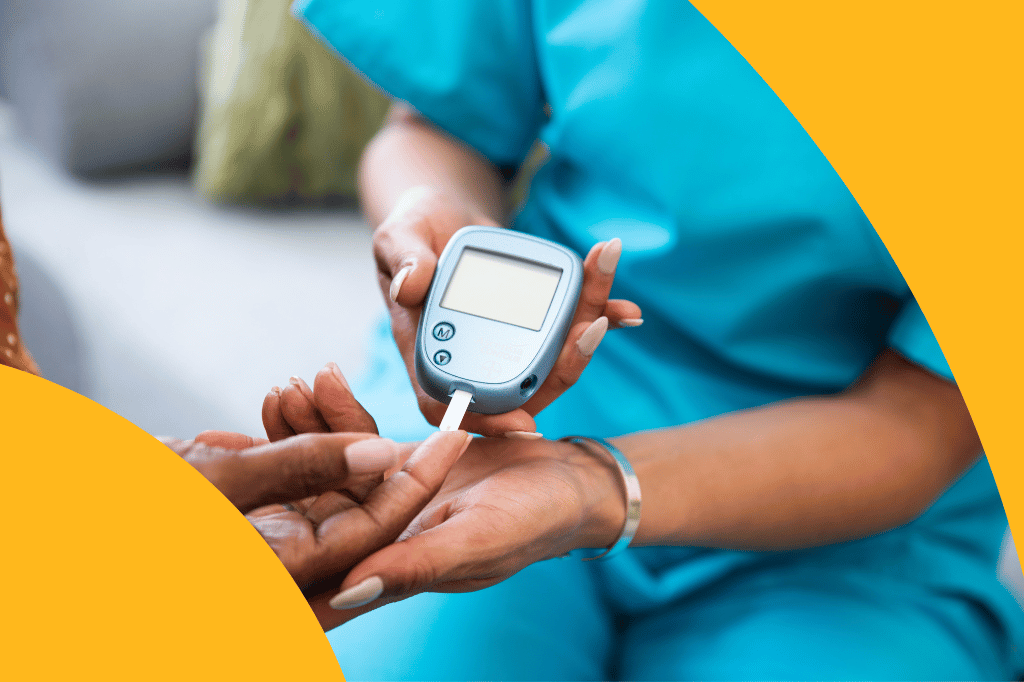
BUILT FOR HEALTHCARE
Ingest, normalize, and blend data
from dozens of health data sources.
Electronic Health Records
Unstructured Clinical Notes
e-Prescribing Data
Vital Signs
Remote Monitoring Data
Medical Claims
Rx Claims
ADT Records
Lab Test Results
Social Needs Assessments
Social Determinants of Health
Operations & Services
Risk patient will develop metabolic syndrome
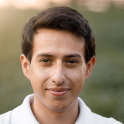
Patient ID
Gender
Age
Risk Score Percentile
583889012
Male
42
97
Impact on risk
Contributing factor
Value
Increase in Fasting Blood Glucose Levels (mg/dL)
80 to 105
BMI Level
29
Rise in Blood Pressure (mmHg)
130/90 to 150/100
Decline in Sleep Quality (episodes / week)
2 to 5
AI INFORMS ACTION
Pinpoint high-risk individuals and surface actionable risk factors.
ClosedLoop generates explainable predictions using thousands of auto-generated, clinically relevant contributing factors.
Provide
Provide patient self-management education
Promote
Promote continuity of care and increase assessment frequency
Improve
Improve adherence to exercise and diet regimes
EXPLORE MORE USE CASES