Predict | Propensity to Pay
Predict propensity to pay and optimize collection.
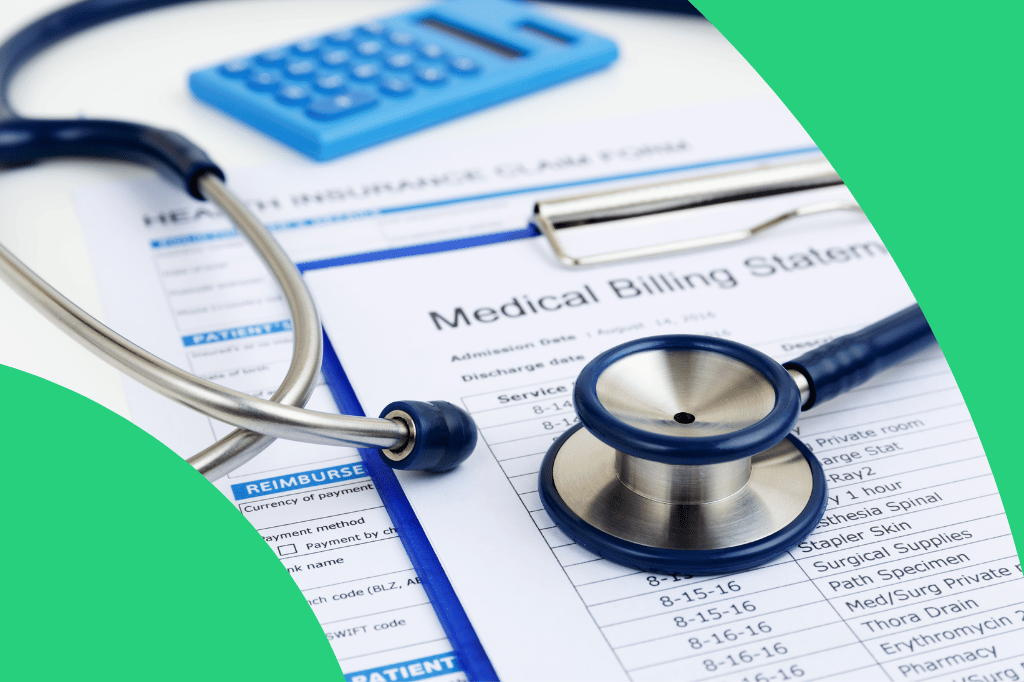
With more than one-third of Americans reporting that an unexpected medical bill greater than $100 would push them into debt, healthcare organizations are grappling with increasing payment collection difficulties, major margin reductions, and a significant loss of revenue. In 2018, $12 million was the average amount of uncompensated care reported by hospitals, and this figure is expected to grow.
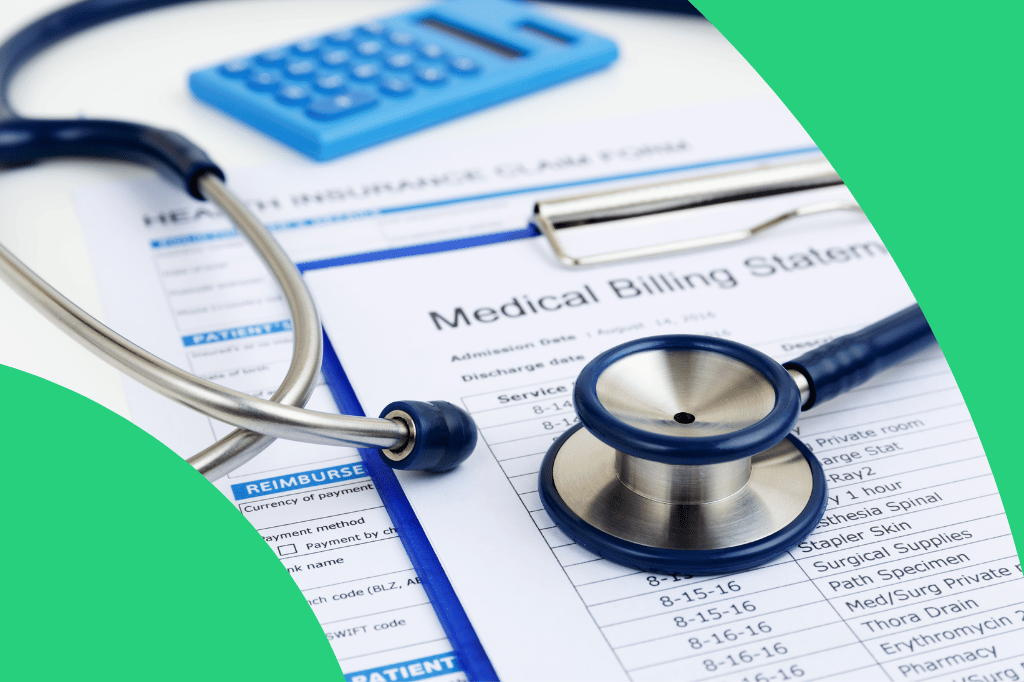
BUILT FOR HEALTHCARE
Ingest, normalize, and blend data
from dozens of health data sources.
Electronic Health Records
Unstructured Clinical Notes
e-Prescribing Data
Vital Signs
Remote Monitoring Data
Medical Claims
Rx Claims
ADT Records
Lab Test Results
Social Needs Assessments
Social Determinants of Health
Operations & Services
Likelihood that patient bill will not be paid in the next six months
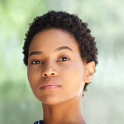
Patient ID
Gender
Age
Risk Score Percentile
239851110
Female
37
98
Impact on risk
Contributing factor
Value
Uninsured Children Percentage Measure
30%
# of Avoidable ER Visits (12M)
3
# of Missed / Rescheduled Appts (12M)
3
Reported Barriers to Care
Transportation
AI INFORMS ACTION
Pinpoint high-risk individuals and surface actionable risk factors.
ClosedLoop generates explainable predictions using thousands of auto-generated, clinically relevant contributing factors.
Support
Support patients with financial literature
Identify
Identify patients likely to qualify for benefits
Direct
Direct patients to appropriate care settings for their unique needs and circumstances
EXPLORE MORE USE CASES
Emergency Room Visits & Observation Stays
Reduce ER visits and enhance continuity of care.