Predict | Serious Fall-Related Injuries
Reduce fall-related injuries and improve functional support.
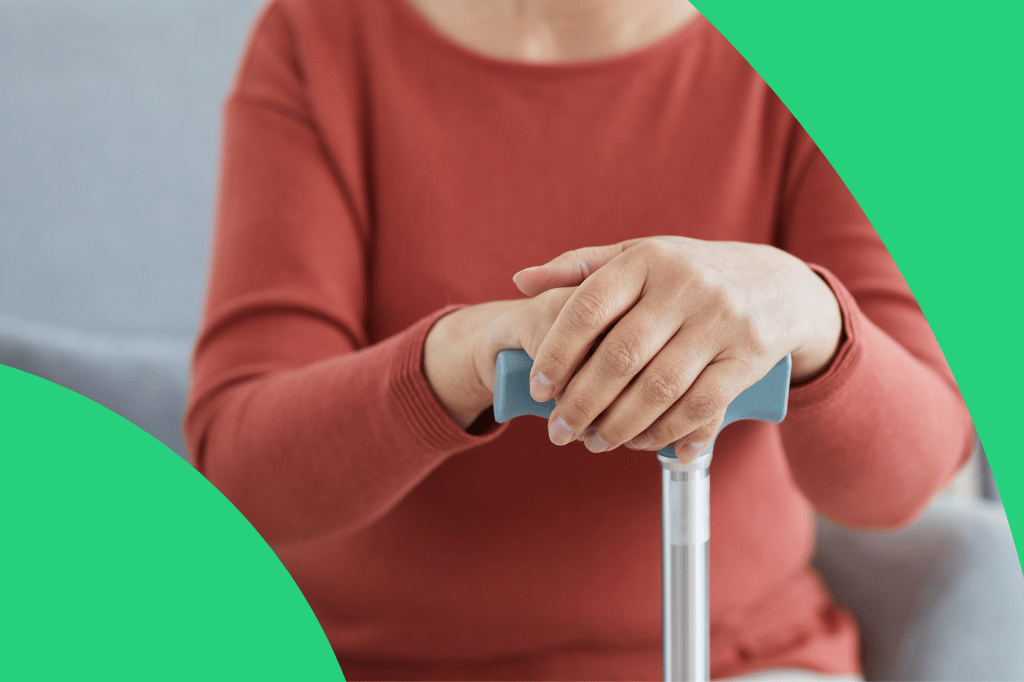
Serious fall-related injuries profoundly affect the lives of older adults, and falls are the leading cause of fatal and nonfatal injuries among older adults. One in four older adults falls each year and nearly 36 million falls were reported in 2018, resulting in more than 900,000 hospitalizations, 32,000 deaths, and $50 billion in healthcare costs—all of which will rise as the population ages.
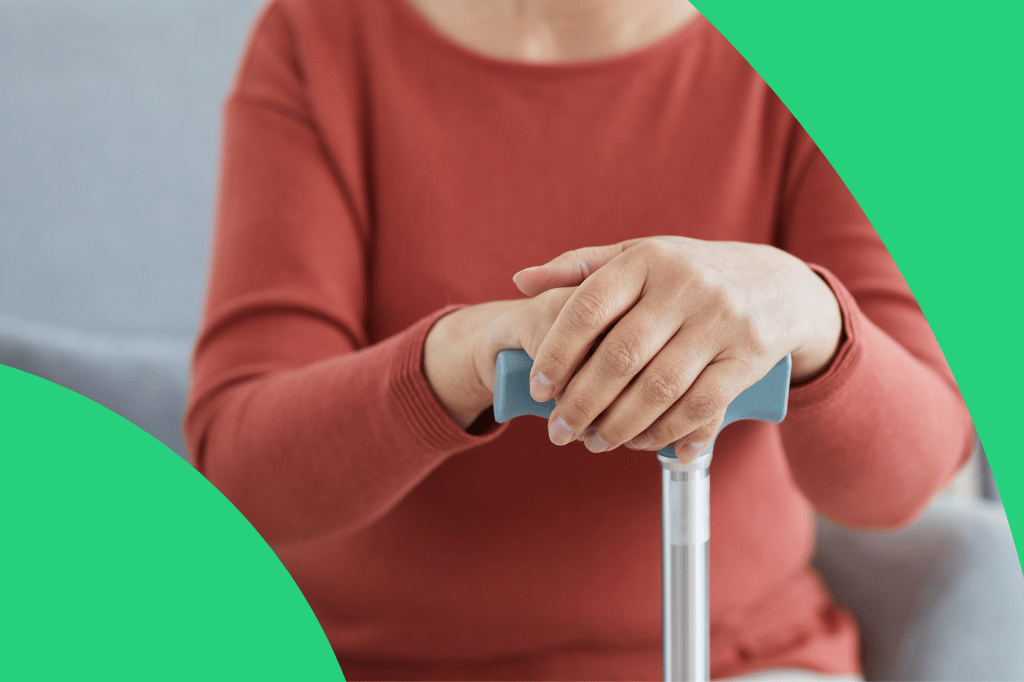
BUILT FOR HEALTHCARE
Ingest, normalize, and blend data
from dozens of health data sources.
Electronic Health Records
Unstructured Clinical Notes
e-Prescribing Data
Vital Signs
Remote Monitoring Data
Medical Claims
Rx Claims
ADT Records
Lab Test Results
Social Needs Assessments
Social Determinants of Health
Operations & Services
Risk of a serious fall-related injury in the next 12 months
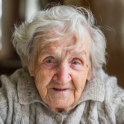
Patient ID
Gender
Age
Risk Score Percentile
712409425
Female
90
98
Impact on risk
Contributing factor
Value
Frailty Percentile
75%
Decline in "Timed Up & Go" Test (sec)
20 to 30
# of Medications on PIM List
1 to 2
# of Fall-related Injuries (12M)
1
AI INFORMS ACTION
Pinpoint high-risk individuals and surface actionable risk factors.
ClosedLoop generates explainable predictions using thousands of auto-generated, clinically relevant contributing factors.
Provide
Provide strength and flexibility training
Employ
Employ assistive devices and increase access to rehabilitation services
Conduct
Conduct medication review, home modification, and post-hospital surveillance
EXPLORE MORE USE CASES
Admission to a Skilled Nursing Facility
Predict SNF admission risk and reduce rehospitalization.
Emergency Room Visits & Observation Stays
Reduce ER visits and enhance continuity of care.