Predict | Unplanned Hospital Admissions
Reduce unplanned hospital admissions and cut costs.
Unplanned Hospital Admissions (UHAs) are acute clinical events that impact approximately 8.5 million Medicare beneficiaries each year. These events are frequently life-altering. For older adults who are hospitalized, 30% experience a significant enough decline in their ability to perform daily activities that it reduces their ability to maintain independence. And for many, that decline is permanent.
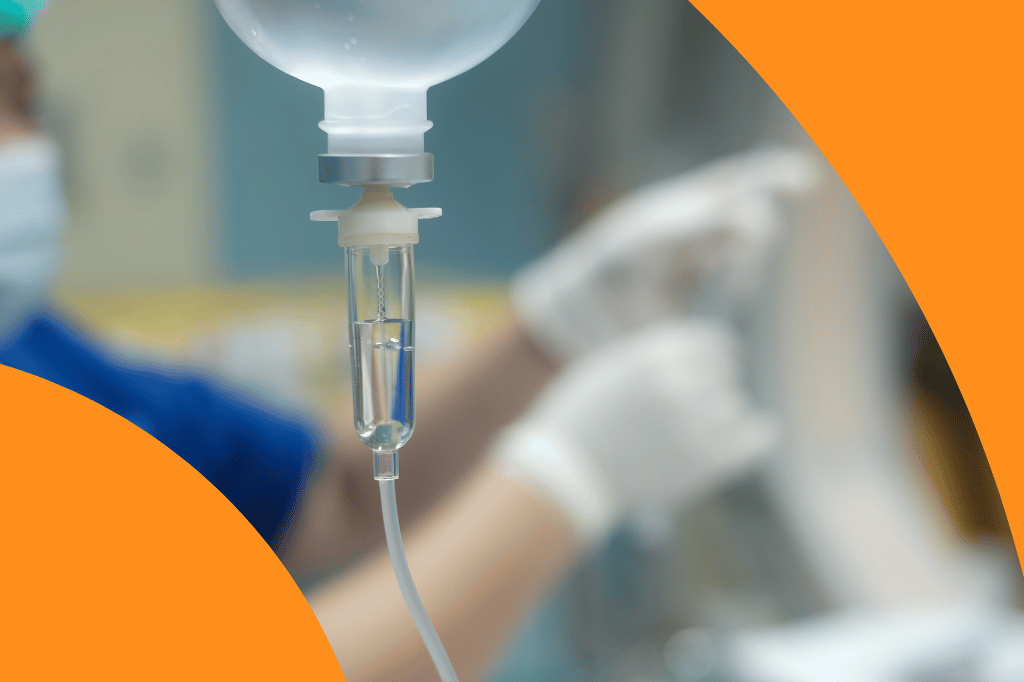
BUILT FOR HEALTHCARE
Ingest, normalize, and blend data
from dozens of health data sources.
Electronic Health Records
Unstructured Clinical Notes
e-Prescribing Data
Vital Signs
Remote Monitoring Data
Medical Claims
Rx Claims
ADT Records
Lab Test Results
Social Needs Assessments
Social Determinants of Health
Operations & Services
Risk of unplanned hospital admission in the next six months
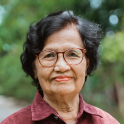
Patient ID
Gender
Age
Risk Score Percentile
746214357
Female
75
96
Impact on risk
Contributing factor
Value
Increase in # of HCCs (12M)
4 to 7
Increase in Delirium Risk Index
3 to 5
# of Days Since Last PCP Visit
375
Pct Flu Vaccination Measure
0.35
AI INFORMS ACTION
Pinpoint high-risk individuals and surface actionable risk factors.
ClosedLoop generates explainable predictions using thousands of auto-generated, clinically relevant contributing factors.
Enhance
Enhance enrollment in chronic care management
Provide
Provide additional support for at-risk patients following planned procedures
Intensify
Intensify care transition planning to potentially avoid readmissions
EXPLORE MORE USE CASES
Avoidable Emergency Department Utilization
Reduce avoidable ED utilization and promote care continuity.