Best Practices for Predicting Member Churn
Discover a few best practices that can help you attempt to predict member churn.
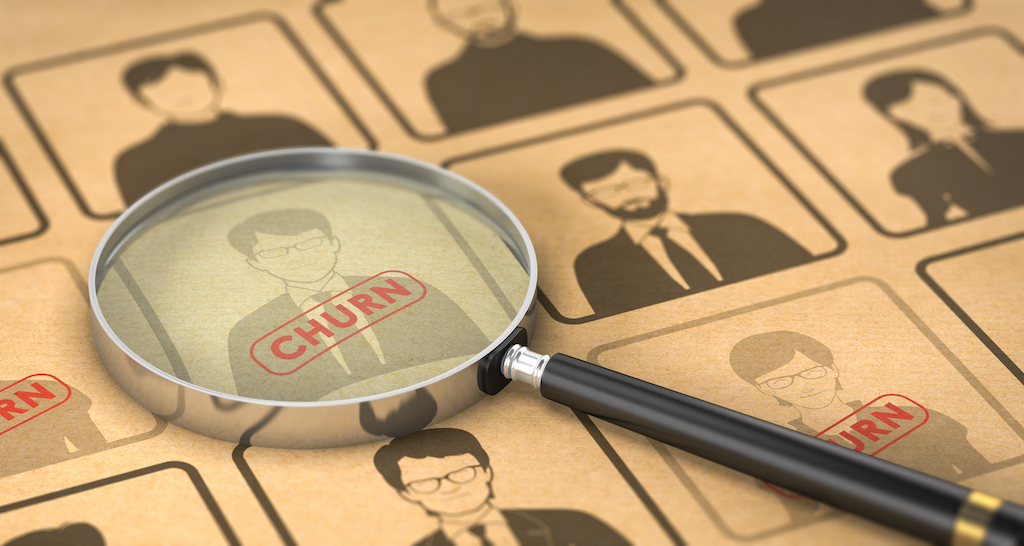
We use cookies to help you navigate efficiently and perform certain functions. You will find detailed information about all cookies under each consent category below.
The cookies that are categorized as "Necessary" are stored on your browser as they are essential for enabling the basic functionalities of the site. ...
Necessary cookies are required to enable the basic features of this site, such as providing secure log-in or adjusting your consent preferences. These cookies do not store any personally identifiable data.
Functional cookies help perform certain functionalities like sharing the content of the website on social media platforms, collecting feedback, and other third-party features.
Analytical cookies are used to understand how visitors interact with the website. These cookies help provide information on metrics such as the number of visitors, bounce rate, traffic source, etc.
Performance cookies are used to understand and analyze the key performance indexes of the website which helps in delivering a better user experience for the visitors.
Advertisement cookies are used to provide visitors with customized advertisements based on the pages you visited previously and to analyze the effectiveness of the ad campaigns.
Discover a few best practices that can help you attempt to predict member churn.
Every year health plans and providers spend a lot of time, resources, and cash fighting to retain their members. There are actually conferences specifically focused on member engagement and retention, allowing organizations to learn tips and tricks from industry leaders.
One strategy for member retention is asking the question “who is likely to disenroll from my plan.” Predicting member disenrollment (“churn”) is an extremely common use case in any member population in which plan choice is an individual’s decision – I’ll explain why below.
Let me set the stage.
Each year 11% of Medicare Advantage plan enrollees voluntarily switch to another plan (Kaiser Family Foundation). For the sake of round numbers, let’s make that 10%. To illustrate what a 10% disenrollment rate actually means to a plan’s revenue, pretend you have 50,000 members and you average $700 per member per month ($8400 per member per year) in payments and premiums. If your organization shares the national average of 10% member churn, that means 5,000 members will opt to leave your plan – losing you $42M in reimbursements. Yes, you read that right – $42M!
Retaining 100% of your members year after year is unrealistic, but what if you could reduce your disenrollment rate from 10% to 9%? How much of an impact does 1% of your population have on your plan’s revenue?
When you reduce your member churn by 1% you retain $4.2M in revenue per year!
With 1% having such a big impact, health plans and providers are understandably looking for solutions to identify which members are most likely to disenroll from their plan. Members that are identified as “high-risk” can be enrolled into incentive/intervention programs in hopes to change the predicted outcome.
If you are looking to build this model in house or are wanting to work with a predictive analytics solution, we’ve found a few best practices that we hope you keep in mind as you attempt to predict member churn.
In his machine learning course at Coursera, Andrew Ng explains when building a new machine learning model you should try and get to a first result as fast as possible, ideally within 24 hours. And we agree!
It’s all about demonstrating potential ROI. Grab your available data sources and get to a first result that will serve as a baseline predictive model. Often we see teams trying to build the most sophisticated model right out of the gate. But what if there’s not enough signal in your data to predict the outcome you want? Start with a light weight model to see what the initial predictions look like. Quickly getting to a baseline model using only your readily available data streams can help demonstrate if there’s enough ROI and determine the value in additional efforts to increase model accuracy.
For member churn specifically, start with a years worth of claims and admissions data. These data sources alone should yield a simple yet insightful baseline model. It might not be as accurate as you ideally want, but it will be able to identify the extremely high-risk members and prove if more time should be spent developing the model deeper.
Communicating ROI looks different when speaking with your Director, VP of Population Health, CFO, or CEO. To assist in conveying the clear value your predictive model can have to your organization, ClosedLoop has an ROI graph built right into the platform. There are a number of different views that tailor the messaging depending on who your audience is.
Machine learning extracts useful patterns from multiple data sources to build a more accurate and comprehensive risk-score. Once you have a baseline model established, start testing the predictive value of additional patient linkable data (ADT feeds, EMR, social factors, labs, etc.). The one line takeaway here – use all available patient data.
This is where the process becomes very expensive and labor intensive if you’re planning to build this model in house. First, think about how many different internal departments and external organizations your data comes from, then think about how the data in each source is formatted in its own specific way. Take the Patient ID column in your claims for example. It’ll be located in one of the hundreds of columns in your data set. Now, look at your ADT feed or at your EHR, not only is this patient ID not located in the same column, but it might have a different column title altogether. If that’s not confusing enough, there’s also a Patient ID column, but it’s not the same ID as in your claims. Manually searching through hundreds of columns in multiple data sources is time consuming, confusing, and no data scientist wants to it!
ClosedLoop has a number of different features built into the platform that automates the process of ingesting disparate data sources, including custom proprietary data sets. In this automated approach, testing and experimenting which data sources yield incremental predictive value requires minimal effort. Since the effort is minimal, try testing as many data sources as you can!
A few of the features include automated data dictionary creation, auto-detection of data types, and auto-clean support for common healthcare elements including diagnosis, procedure, and drug codes.
When predicting member churn, we’ve found a couple external data sources that can increase model accuracy. For example social determinants data such as the USDA Food Atlas and the Census data (which are also public!) can provide a lot of value by giving you a picture of the nutritional and economical landscape of your population. Now, starting with these data sources on their own would not yield very good results (and it’s extremely tricky to do), but layering them on top of a baseline model containing claims with patient demographic data is highly effective. The more data sources that power your predictive model, the more comprehensive and explainable your risk-scores can be.
Great, you’ve identified the at-risk members. But, predicting who is most likely to disenroll from your plan is only the first piece of the puzzle. What are you going to do with the predictions?
Attaching an intervention to at-risk members is an opportunity for your organization to take action and attempt to change the predicted outcome. But what should your intervention be? It might be calling high-risk members to ask if they liked their physician and if not, offer to schedule their next appointment with a new one. Maybe it’s a real phone call reminder from a care manager, rather than from an automated line. For members going out of network, the intervention could be to suggest referrals for in-network providers.
At ClosedLoop, we suggest leveraging the analytics in multiple ways. Again, if you’re planning to build this model in house, this piece is quite tricky. Offering multiple interventions can be extremely beneficial by proving similar groups of patients with an individualized care approach, but how do you decide which patient would benefit most from which intervention?
When targeting specific cohorts of patients for different interventions, it helps to know the reason why a member was identified as high-risk. ClosedLoop provides just that, patient-level explainability factors. These factors allow you to see the exact reasons why someone was identified as high-risk making it easier to associate specific cohorts of members to different interventions.
Predicting Member churn is top of mind for all health plans which member choice is an individual’s decision, and after seeing the impact 1% of your members can have, it’s easy to see why. If you are looking to either build this predictive model or work with a solutions provider, we hope you remember these best practices.
There’s a lot to think about when building a predictive model for member churn. Luckily, we’ve already built this model at ClosedLoop and it’s listed on our catalog of off-the-shelf models.
If you want to speak with an expert to brainstorm ways your organization can leverage this particular model (or others), please reach out and a member of our team will be happy to chat with you.
Interested in learning more about building models for Healthcare? Check out these related posts for some practical insights from members of our team:
CONTINUE READING
Artificial intelligence (AI) and machine learning (ML) are increasingly used in healthcare to combat unsustainable spending and produce better outcome...
13 min read
Implementing a comprehensive strategy to advance health equity is a moral and financial imperative for healthcare organizations (HCOs). Persistent...
12 min read
COVID-19 simultaneously exacerbated existing health disparities and introduced entirely new ones. The pandemic disproportionately impacted people of c...
11 min read
Get in touch today to see the ClosedLoop platform in action.
Notifications