Predict | Drug Safety
Anticipate and avoid adverse drug reactions.
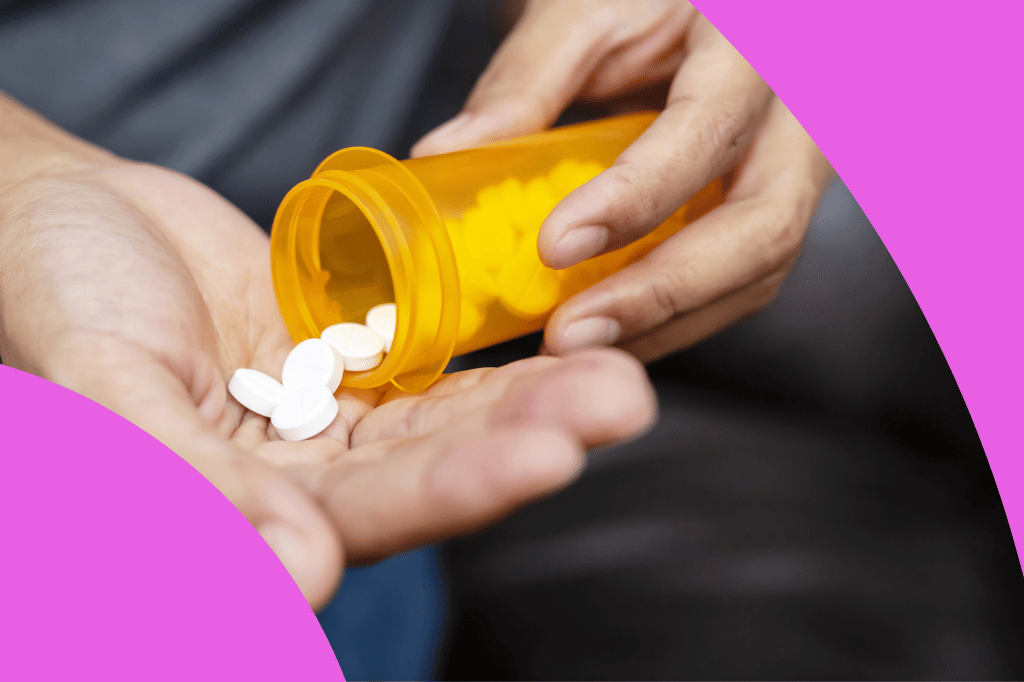
Every year, Adverse drug reactions (ADRs) – unintended, harmful events attributed to intended medicine use – result in more than 750,000 inpatient injuries or deaths, affect nearly two million hospital stays, and directly result in over one million ED visits and 125,000 hospitalizations. ADRs are estimated to cause at least 10% of all admissions in older adults.
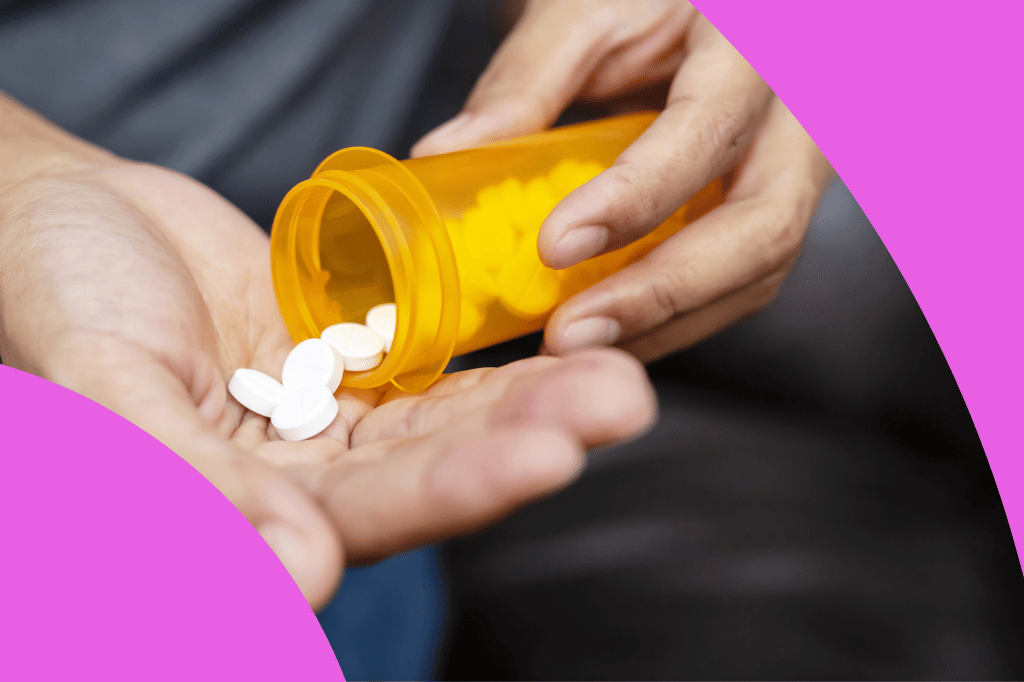
BUILT FOR HEALTHCARE
Ingest, normalize, and blend data
from dozens of health data sources.
Electronic Health Records
Unstructured Clinical Notes
e-Prescribing Data
Vital Signs
Remote Monitoring Data
Medical Claims
Rx Claims
ADT Records
Lab Test Results
Social Needs Assessments
Social Determinants of Health
Health Risk Assessments
Risk of serious fall-related injury in the next 12 months
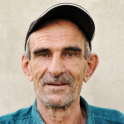
Patient ID
Gender
Age
Risk Score Percentile
989621109
Male
76
98
Impact on risk
Contributing factor
Value
Change in Anticholinergic Cognitive Burden Scale
1 to 5
Increase in # of Unique Prescribers
5 to 7
Frailty Percentile
0.70
Level of Reported Medication Side Effects
High
AI INFORMS ACTION
Pinpoint high-risk individuals and surface actionable risk factors.
ClosedLoop generates explainable predictions using thousands of auto-generated, clinically relevant contributing factors.
Enhance
Enhance medication reconciliation and de-prescribing efforts
Ensure
Ensure safe use proactively in regular appointments
Educate
Educate patients about drug safety and regularly assess patient condition
EXPLORE MORE USE CASES
Admission to a Skilled Nursing Facility
Predict SNF admission risk and reduce rehospitalization.