Predict | Rising Risk and Cost Bloomers
Identify rising risk individuals and improve outcomes.
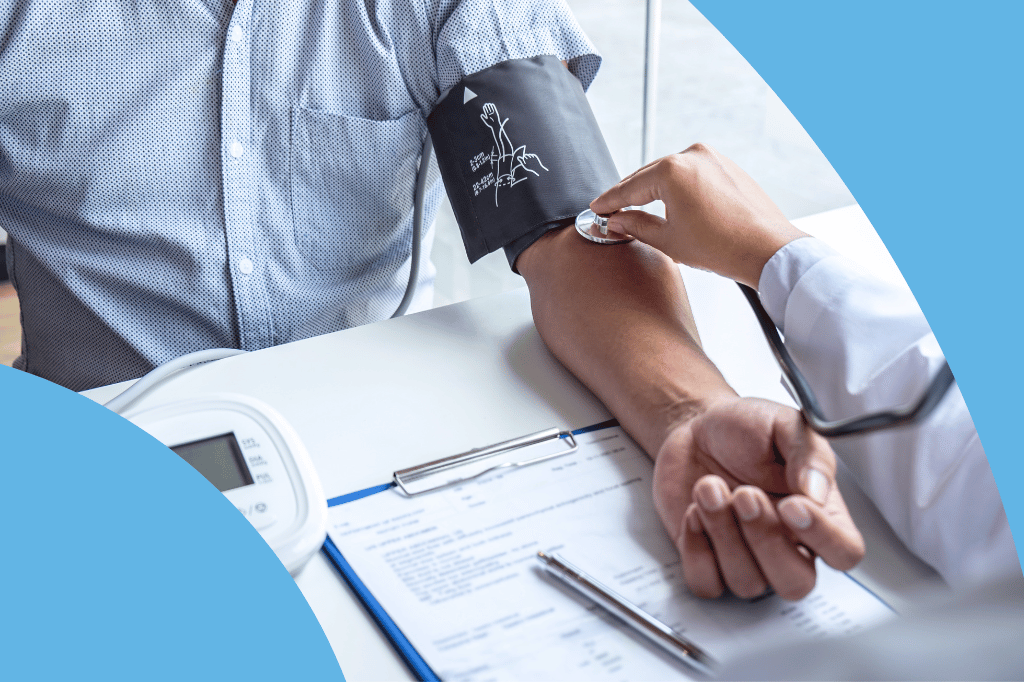
The costliest 10% of patients account for more than two-thirds of healthcare spending. While some of these high-cost patients have consecutively high-cost years, the majority experienced a ‘cost bloom’—a dramatic year-over-year cost increase that catapulted them into the upper decile of spending. These previously low-cost patients account for roughly 68% of all high-cost patients annually.
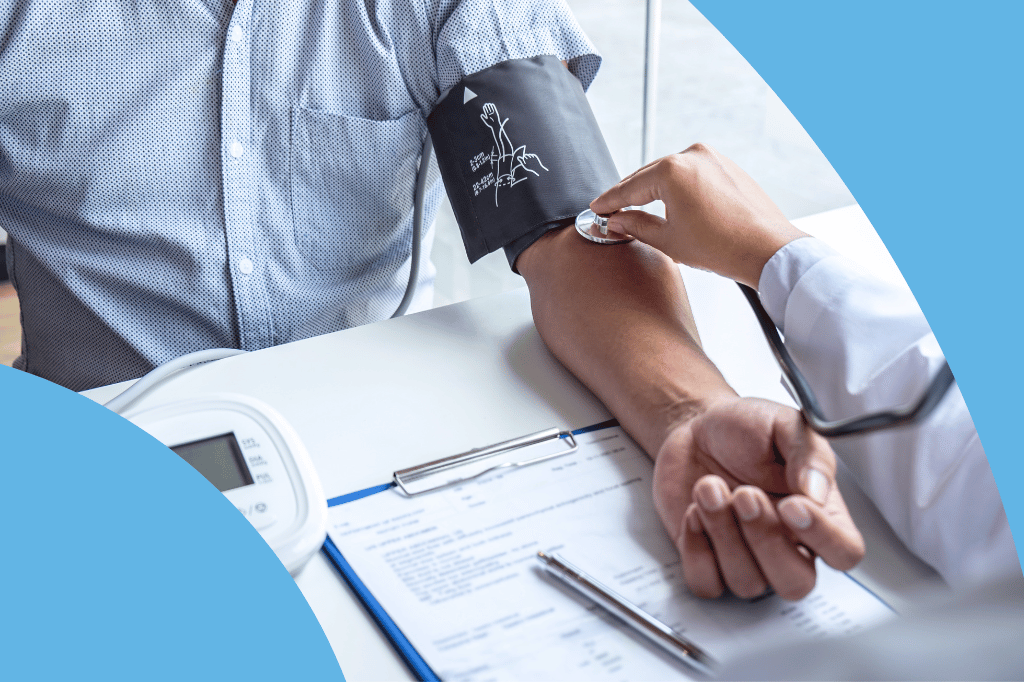
BUILT FOR HEALTHCARE
Ingest, normalize, and blend data
from dozens of health data sources.
Electronic Health Records
Unstructured Clinical Notes
e-Prescribing Data
Vital Signs
Remote Monitoring Data
Medical Claims
Rx Claims
ADT Records
Lab Test Results
Social Needs Assessments
Social Determinants of Health
Operations & Services
Risk of costs rising at least 50% in the next 12 months
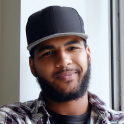
Patient ID
Gender
Age
Risk Score Percentile
441769021
Male
48
97
Impact on risk
Contributing factor
Value
# of Distinct Providers (12M)
10
Percentage Abnormal Lab Results
45%
Resting Heart Rate
90
Total # of Lab Tests (3M)
20
AI INFORMS ACTION
Pinpoint high-risk individuals and surface actionable risk factors.
ClosedLoop generates explainable predictions using thousands of auto-generated, clinically relevant contributing factors.
Encourage
Encourage strong continuity of care
Promote
Promote early identification of chronic condition development
Increase
Increase screening and assessment frequency for at-risk patients
EXPLORE MORE USE CASES