Predict | Suspect HCCs
Identify suspect HCCs and improve accuracy.
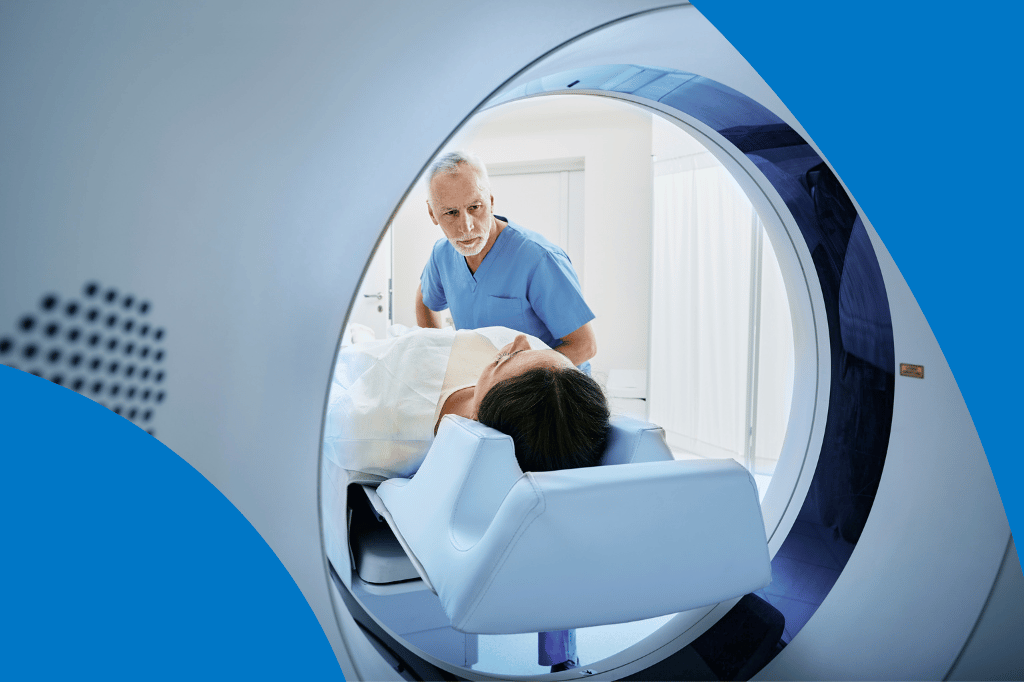
Risk adjustment is quietly becoming an economic cornerstone in healthcare, and the Hierarchical Condition Category (HCC) is at its core. Because HCCs determine payments, they influence an organization’s economic viability, available resources, and care delivery capacity, which means accurate and complete diagnosis coding is becoming an economic and clinical imperative.
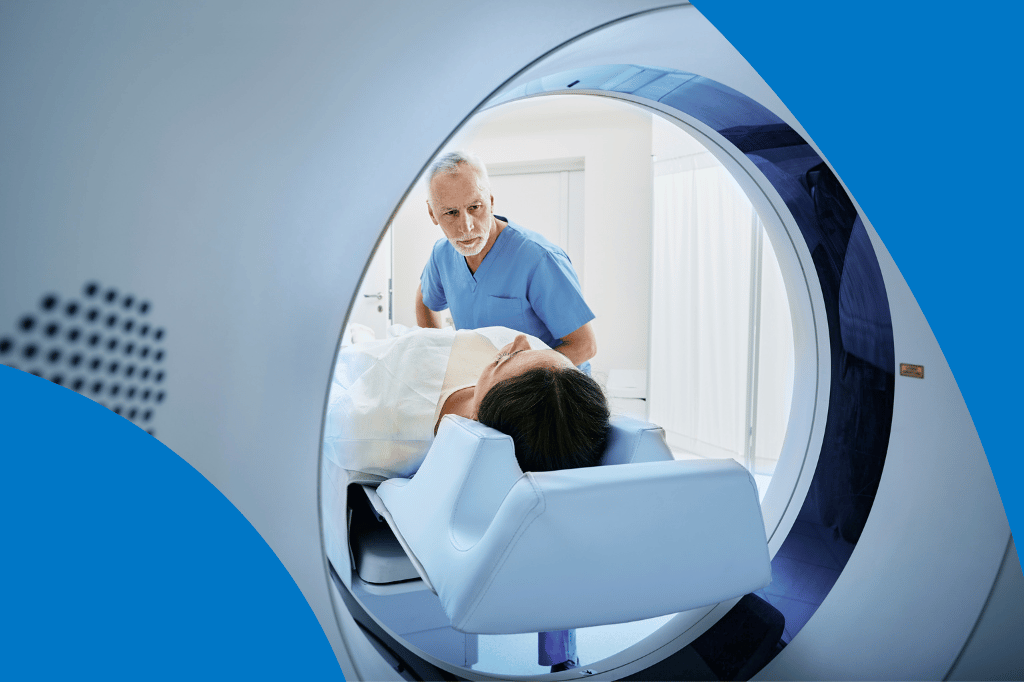
BUILT FOR HEALTHCARE
Ingest, normalize, and blend data
from dozens of health data sources.
Electronic Health Records
Unstructured Clinical Notes
e-Prescribing Data
Vital Signs
Remote Monitoring Data
Medical Claims
Rx Claims
ADT Records
Lab Test Results
Social Needs Assessments
Social Determinants of Health
Operations & Services
Likely undocumented condition for CHF
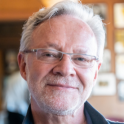
Patient ID
Gender
Age
Risk Score Percentile
143958712
Male
71
97
Impact on risk
Contributing factor
Value
Decline in LV Ejection Fraction
0.45 to 0.35
Dyspnea on Exertion
June 14, 2020
Procedure for Electrocardiogram
June 14, 2020
e-Rx for Beta Blockers
June 14, 2020
AI INFORMS ACTION
Pinpoint high-risk individuals and surface actionable risk factors.
ClosedLoop generates explainable predictions using thousands of auto-generated, clinically relevant contributing factors.
Identify
Identify and address gaps in coding to capture annual capitation payments
Assess
Assess documentation determinations to automatically improve accuracy
Refine
Refine diagnosis identification continuously
EXPLORE MORE USE CASES
Adverse Glycemic Events & Continuous Glucose Monitoring
Improve glycemic control and avoid adverse outcomes.