Predict | Transitions of Care
Improve transitions of care and reduce readmissions.
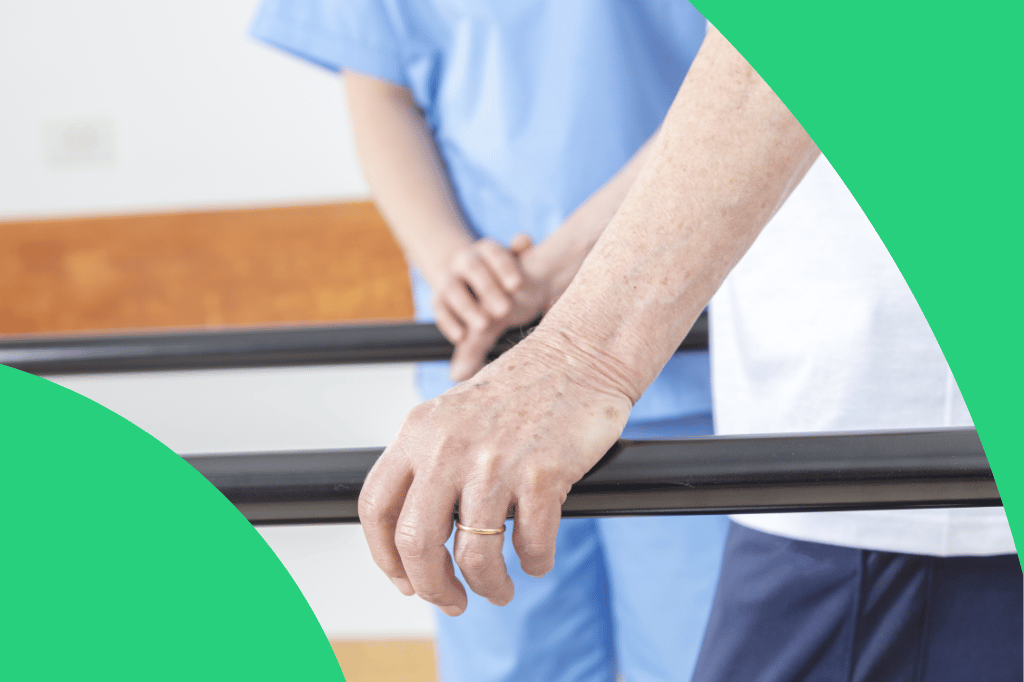
Being discharged from the hospital can be bad for your health. Nearly one in five adult patients experience an adverse event within three weeks of leaving the hospital, and roughly 20% of Medicare patients discharged from a hospital—approximately 2.6 million older adults—are rehospitalized within 30 days, at a cost of over $26 billion every year.
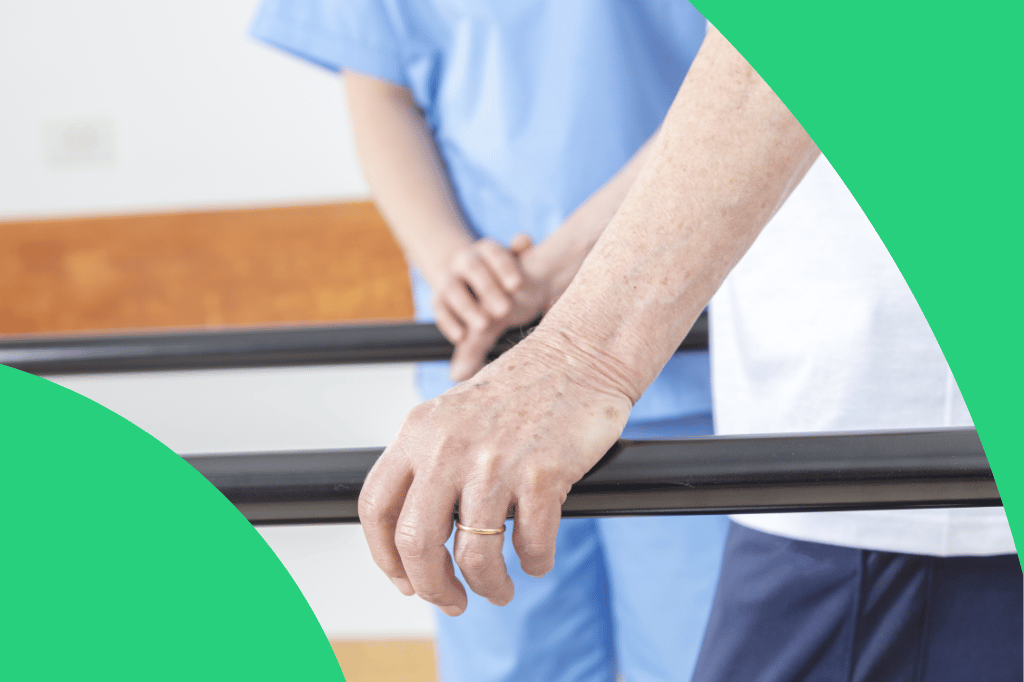
BUILT FOR HEALTHCARE
Ingest, normalize, and blend data
from dozens of health data sources.
Electronic Health Records
Unstructured Clinical Notes
e-Prescribing Data
Vital Signs
Remote Monitoring Data
Medical Claims
Rx Claims
ADT Records
Lab Test Results
Health Risk Assessments
Social Determinants of Health
Operations & Services
Risk of poor care transition and unplanned admission in the next 30 days
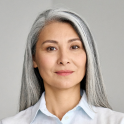
Patient ID
Gender
Age
Risk Score Percentile
129093652
Female
73
96
Impact on risk
Contributing factor
Value
# of Unplanned Admissions (12M)
1
Hemoglobin (g/dL)
11
# of ER Visits (6M)
2
Level of Social Support
Low
AI INFORMS ACTION
Pinpoint high-risk individuals and surface actionable risk factors.
ClosedLoop generates explainable predictions using thousands of auto-generated, clinically relevant contributing factors.
Enhance
Enhance patient engagement and self-management education
Promote
Promote consistent caregiver relationships
Improve
Improve medication reconciliation and adherence
EXPLORE MORE USE CASES
Adverse Glycemic Events & Continuous Glucose Monitoring
Improve glycemic control and avoid adverse outcomes.