Predict | Diabetes
Predict high diabetes risk and avoid adverse complications.
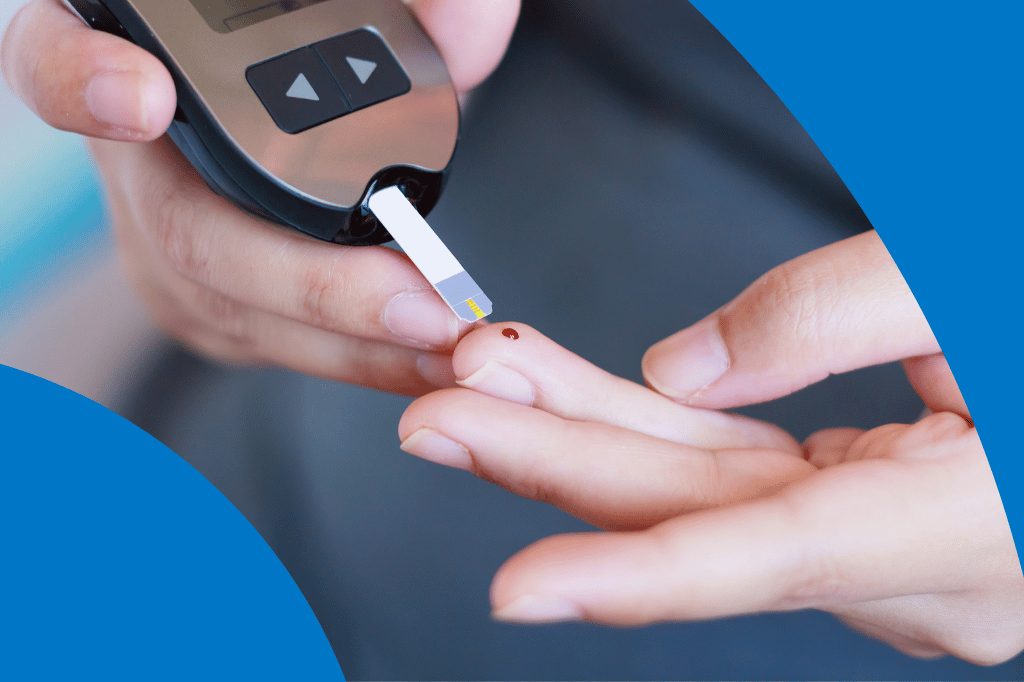
Diabetes affects approximately 34 million adults—more than 10% of Americans—and is the seventh leading cause of death in the United States. In the last 20 years, the number of adults diagnosed with diabetes has more than doubled, and the healthcare costs for diabetes are estimated to exceed $325 billion. Despite this, more than one in five people with diabetes are unaware of their condition.
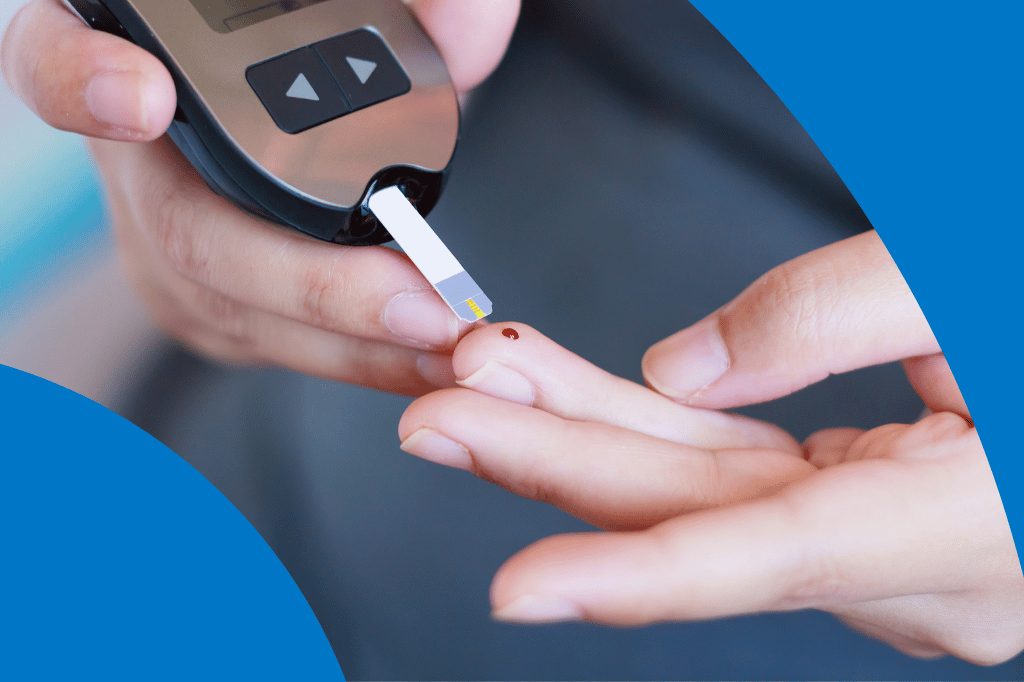
BUILT FOR HEALTHCARE
Ingest, normalize, and blend data
from dozens of health data sources.
Electronic Health Records
Unstructured Clinical Notes
e-Prescribing Data
Vital Signs
Remote Monitoring Data
Medical Claims
Rx Claims
ADT Records
Lab Test Results
Social Needs Assessments
Social Determinants of Health
Operations & Services
Risk of potentially avoidable hospitalization for diabetes in the next 12 months
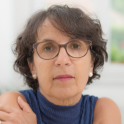
Patient ID
Gender
Age
Risk Score Percentile
127893417
Female
61
94
Impact on risk
Contributing factor
Value
Medicare Disability Status
Disabled
Increase in # of HCCs (12M)
4 to 6
# of Days Since Last PCP Visit
375
Pct Mammography Screening
39%
AI INFORMS ACTION
Pinpoint high-risk individuals and surface actionable risk factors.
ClosedLoop generates explainable predictions using thousands of auto-generated, clinically relevant contributing factors.
Promote
Promote self-monitoring of blood sugar to achieve glycemic control
Encourage
Encourage adherence to healthy dietary practices and regular exercise
Strengthen
Strengthen care continuity and increase assessment frequency
EXPLORE MORE USE CASES