Predict | Frailty
Identify frailty and address functional decline.
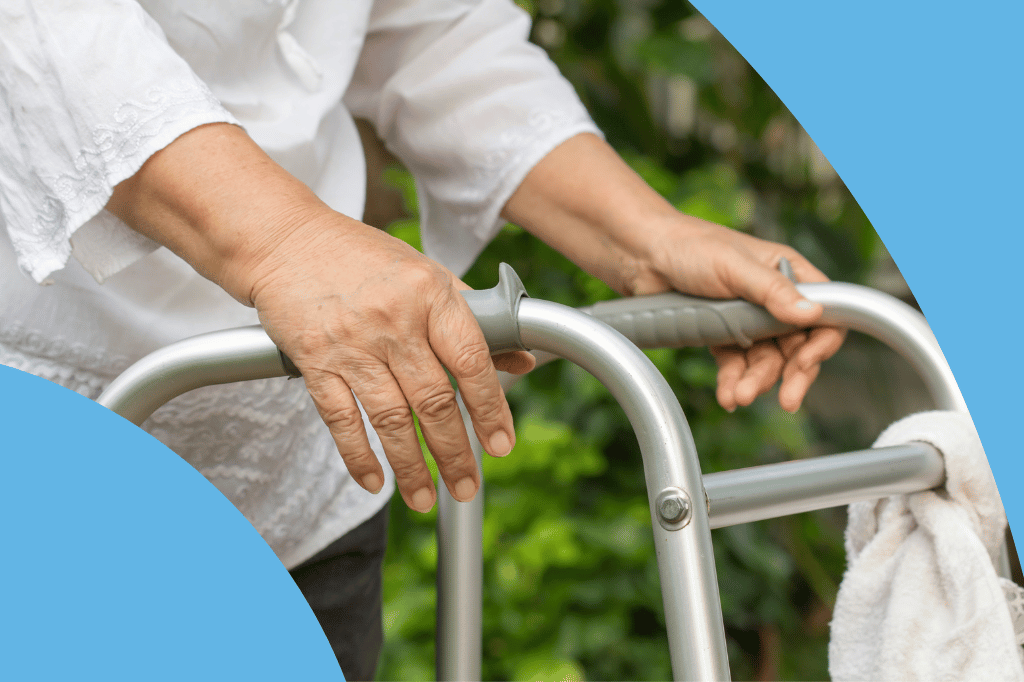
Frailty occurs in approximately 25% of older adults and results in increased vulnerability to poor health outcomes, worsening mobility and disability, hospitalizations, and mortality. For older adults, it doubles the risk of in-hospital mortality and increases the risk of one-year mortality by 50%. It also greatly contributes to cost – estimated at more than $14,000 per patient annually.
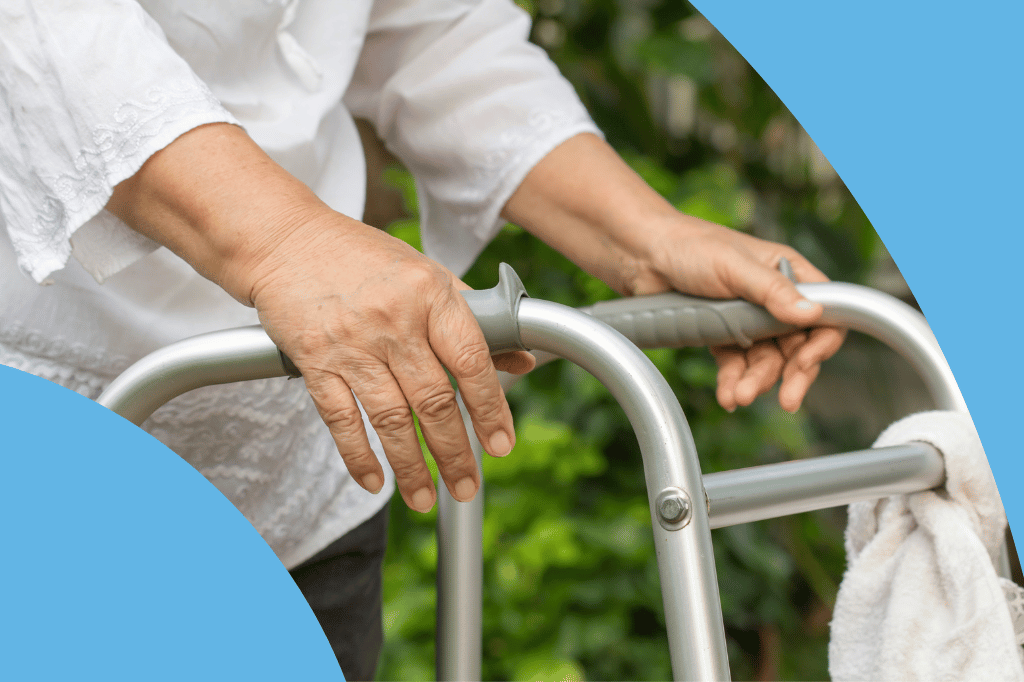
BUILT FOR HEALTHCARE
Ingest, normalize, and blend data
from dozens of health data sources.
Electronic Health Records
Unstructured Clinical Notes
e-Prescribing Data
Vital Signs
Remote Monitoring Data
Medical Claims
Rx Claims
ADT Records
Lab Test Results
Social Needs Assessments
Social Determinants of Health
Operations & Services
Risk of a Serious Fall-Related Injury in the Next 6 Months
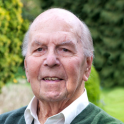
Patient ID
Gender
Age
Risk Score Percentile
710399422
Male
84
95
Impact on risk
Contributing factor
Value
Frailty Score
0.87
# of Unplanned Admissions (12M)
1
# of Units of Durable Medical Equipment (6M)
2 to 5
Levels of Caregiver Support
Low
AI INFORMS ACTION
Pinpoint high-risk individuals and surface actionable risk factors.
ClosedLoop generates explainable predictions using thousands of auto-generated, clinically relevant contributing factors.
Engage
Engage patients in cognitive training activities
Promote
Promote regular physical activity and adherence to self-management programs
Supplement
Supplement patient nutrition and enhance care continuity
EXPLORE MORE USE CASES
Avoidable Emergency Department Utilization
Reduce avoidable ED utilization and promote care continuity.